This case study details how we helped a company that produces rapid chargers for Electric Vehicles (EV) to deploy its products in the UK using a successful data-driven strategy (Location Scoring Solution).
- Location Analytics
- Location Scoring Solution
- Business Intelligence
- Machine Learning
- Cloud Architecture
- Data-driven Decisions
- Energy Industry
- Rapid Chargers
Key challenges
Our client is one of the big and fast-growing companies for electronic vehicle charging in the UK. They provide a rapid charger for people who need to recharge their vehicle for a short period of time, from 30 to 60 minutes, when stopping at a gas station or a grocery store for example.
They want to cover the UK market in the best possible way, offering their users the possibility to be where they need them the most. Currently, they use two methods to identify locations: their team searching for locations based on hunches and potential partners, such as supermarkets or fast-food restaurants who are interested in getting equipped.
In this context, our client was looking to:
- Establish a reliable and effective decision-making process to decide on the most appropriate locations for implementing their chargers in order to maximize the market coverage and utilization of these chargers.
- Decide how many chargers to deploy in each location (the standard number being 2 chargers).
- Allocate less time to the decision process for the implementation of their products.
Our approach
In order to meet our client’s challenges we:
- Took the time to investigate the information that was used in order to identify new one to use;
- Searched for this new data needed through partnerships, public data (traffic, parking availability, points of interest like cinemas, grocery, gyms…) and the existing charging network;
- Set up the entire infrastructure on the client’s AWS cloud environment, built the processing pipeline, the model management and implemented a powerful visualization tool (PowerBI);
- Merged of all public, own, as well as paid data sources in one place, modeled the algorithms and developed the front-end application;
- Organized many workshops to tuned the insights in order to address the clients’ requests in the best way;
- Scheduled all the data and analytics jobs so they can be updated and run alone, providing always up-to-date insights;
- Provided the end-over documentation for our client to be autonomous.
Benefits
- Operationalized Machine Learning model helping to find the best available location for rapid chargers’ implementation.
- Data-driven decision-making based on an intuitive dashboard providing all relevant insights for every location (over 400 data points and forecasts).
- Agile self-service approach enabling business users to adjust and expend the dashboard as they want and get all information at a glance (KPIs, traffic, competition, environment, demographics).
- Full transfer of the Intellectual Property to the company: they own the data and manage the entire solution.
- Very low operating cost: our client realizes savings over time by evolving the use of the solution, compared to existing SaaS solution on the market.
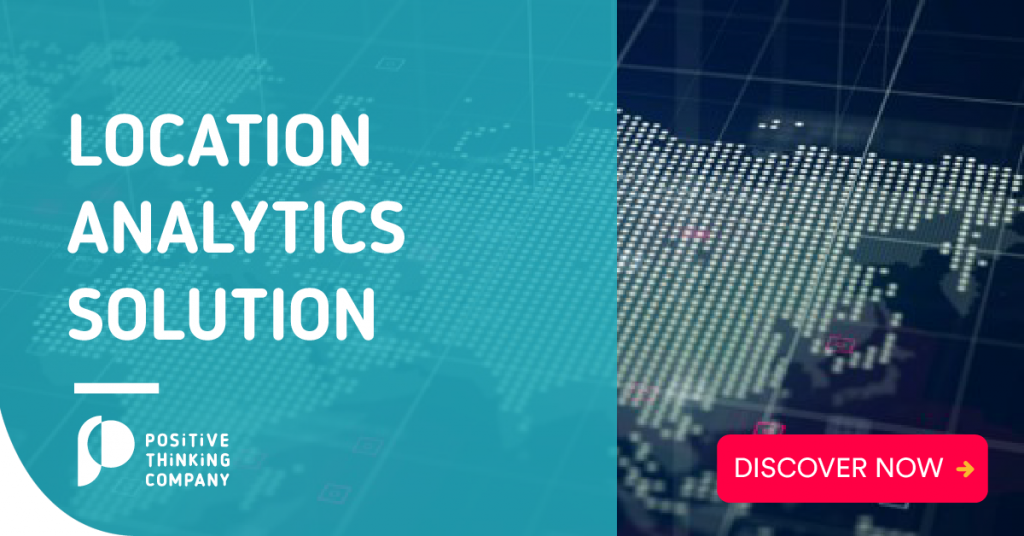
Team involved
Data Scientists, Data Engineers, Cloud Architects and Project Manager collaborated with our client for 3 months.
Technologies & Partners

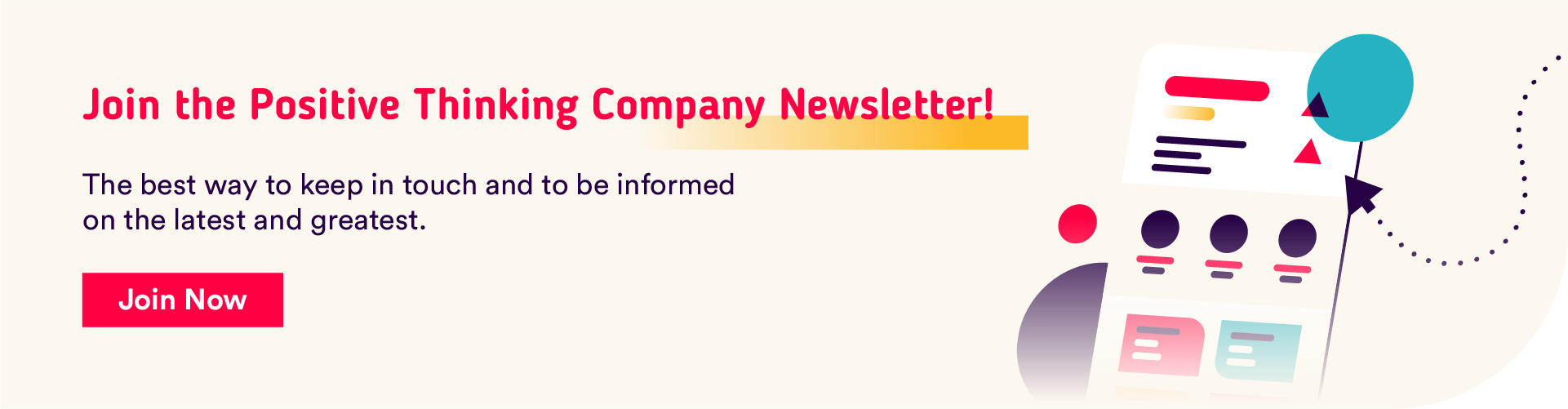