This use case article gives an overview of the Strategic, Technical and Organizational implementation of Data Science at Deutsche Automobil Treuhand (DAT).
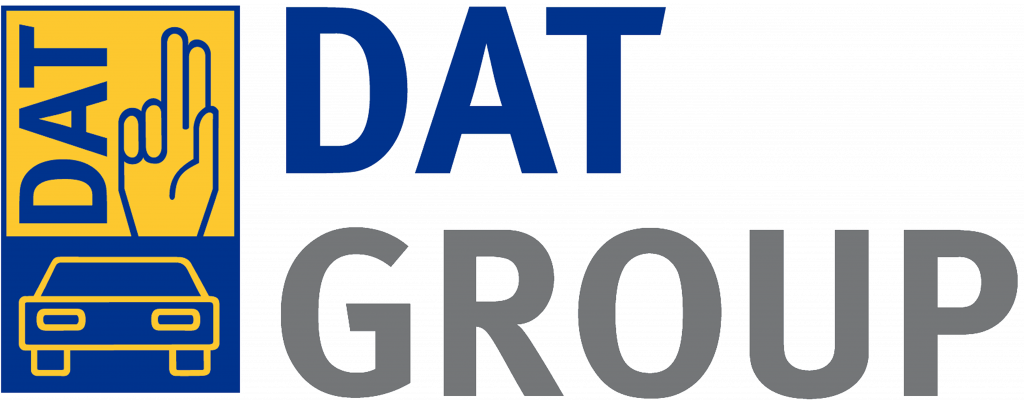
- AI Strategy
- AI Maturity Assessment
- Data Science Platform
- Data-driven organization
- Computer Vision
- Deep Learning
- Image Recognition
Key challenges
Our client is DAT Group, an international company operating in the automotive industry. They provide automotive data, products and services that focus on enabling a digital vehicle lifecycle for over 90 years.
Data being an important part of their core business, they were used to manipulating it, analyze it and in fine take decisions based on it. In their journey to become an even more data-driven company, DAT wanted to innovate by effectively integrating new AI and Data Science capabilities to their business.
Challenges were therefore to :
- Combine the existing know-how with the most innovative methods and technologies
- Set up a data science infrastructure and provide best practices
- Enable them to model unstructured (text, images & videos) and structured data
- Ensure the coordination of all data science initiatives across all business units
Our approach
In order to address DAT’s challenges and enable them to take advantage of advanced analytics capabilities:
1. We helped them define a clear AI strategy:
- AI Maturity Assessment: In-depth analysis, thought workshops, of existing organization, infrastructure, processes, and data.
- Roadmap definition and validation: Derivation of a tailor-made use case driven data science strategy & analytical roadmap.
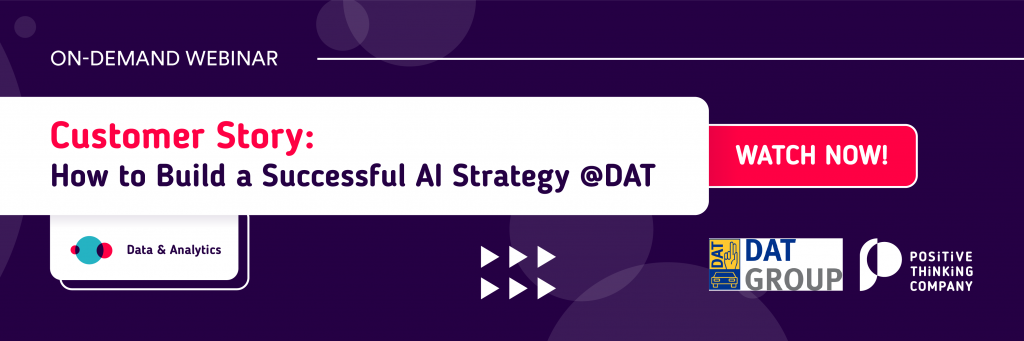
2. We supported them both in defining and setting up an implementation plan:
- Infrastructure: Architecture and installation of a cloud ecosystem as a data science platform.
- Processes: Definition of standardized processes and governance for managing data science solutions.
- Use cases: Development of multiple use cases from POC to production, including for example: text search and annotations using NLU (Natural Language Understanding), building regression models for quality assessments or building and deployment of computer vision models.
- Organization: Internal team building, coaching and trainings In order to sensitize internally and facilitate change management.
Benefits
- Increase efficiency with modern, data-driven methods.
- Centralization and better collaboration thanks to a complete DSP (lab and factory) architecture for model management.
- Standardize and scalable pipelines to fill data analytics data lakes.
- Positive and tangible results thanks to the actual operationalization of machine learning models (not only POC development).
- Next step: Discussions about having a Data Product approach and modernizing the BI strategy.
Team involved
6 experts involved during 18 months:
- Project Manager
- Product Owner
- Data Scientist
- Deep Learning Engineer
- ML Engineer
- Reporting Engineer
Technology & Partner

Technologies used for this use case: Python, Azure Machine Learning, Azure Cloud, Apache Spark, Data Bricks and ML Flow