This case study details how we helped a European telecommunications company to develop its own Virtual Assistant and to industrialize its Natural Language Understand (NLU) activities. From putting NLU at the center of their project to implementing new features and the proper management of the NLU activities, we collaborated with our client’s teams for more than two years.
Key Challenges
Our client is one of the largest telecommunications providers in Europe. They provide fixed-network, mobile communications and internet to millions of individuals and information and communication technology solutions to business organizations.
Convinced that Voice technologies are the next big trend in the Telcos sector, our client invested in the development of a Smart Speaker and an embedded Virtual Assistant. Their goal was obviously to generate net new revenues and to tackle this new market as a European pioneer. In fact, they were partnering with another Telecommunications leader to launch a product with a strong competitive advantage: being compliant by design with the GDPR regulation.
The Smart Speaker had successfully been developed, but our client was struggling with the conception of the Virtual Assistant. Several teams were working on this new product, but they were lacking key skills and techniques in Natural Language Processing (NLP) in general and in Natural Language Understanding (NLU) specifically. In fact, they had developed a first version of the NLU component that did not meet their expectations.
Therefore, our client was looking to:
- Get expert advice and guidance on establishing and applying the right NLU strategy;
- Industrialize the NLU activities with regard to the best practices;
- Improve existing features and create new ones.
They thought building the hardware was the hardest part, but that was before developing the Virtual Assistant.
NLU Consultant at Positive Thinking Company
Our Approach
The team of our client was composed of very experienced developers and data scientists, but with very little knowledge and experience in language data, NLP use cases in general and NLU specifically. Having this kind of set of skills and expertise was actually a key success factor for this very complex project.
By organizing workshops with the team and the product owners we assessed the current maturity of the project (with regards to NLU). We quickly identified the core dimensions of the project to focus on: creating awareness on the importance of the NLU aspect, professionalizing the NLU activities, getting the Virtual Assistant features to the next level and ensuring the proper management of the advanced analytics capabilities development.
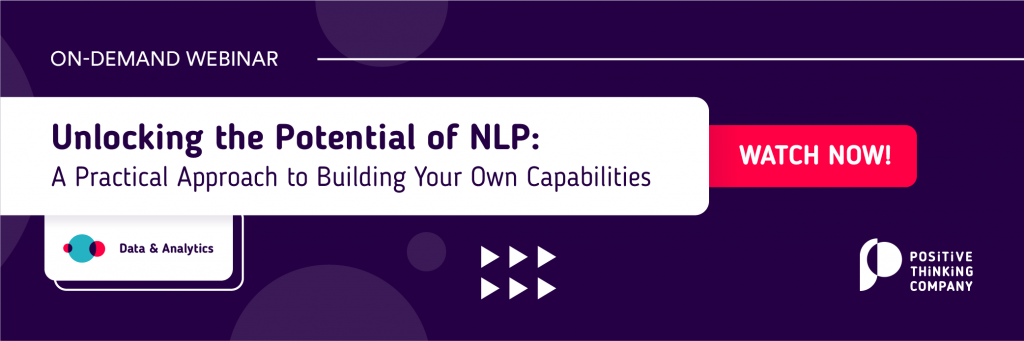
Putting the NLU at the center of the project
- Creating awareness about the importance of the NLU in a voice technology project via documentation, trainings and Q&A sessions handled by experienced consultants;
- Increasing the team of NLP and NLU experts, supporting the recruiting of new experts and conception and implementation of their onboarding;
Professionalizing the NLU activities
- Strong expansion of data annotation activities by increasing the members of the annotation team, staying in close exchange with them and by equipping them with a professional annotation tool;
- Initiation and introduction of a dedicated in-house data annotation tool and a database to manage the labeled data in a meaningful way (always respecting data privacy and protection in the sense of GDPR);
- Migrating from the actual NLU data provider to a better one to ensure quality and proper training of the developed ML models;
- Introduction of the versioning of language models by designing a complete NLU Model lifecycle for the management of modifications and updates (as part of our MLOps best practices);
- Automation of NLU activities to make the modification of the NLU much more efficient;
- Addressing ambiguity issues (as utterances can have more than one meaning) by proposing a dedicated solution;
- Defining and proposing data governance rules and responsibilities for accessing the data (in order to be fully GDPR compliant).
Improvement of existing and implementation of new Virtual Assistant features
- Revising the existing language models to make them “linguistically future-proof” and to achieve good recognition rates (e.g. radio, weather, TV, smarthome, alarm, reminder);
- New Smarthome features (e.g. changing the light temperature and color, opening window blinds)
- Seasonal features (e.g. advent calendar)
- Clock features (e.g. reminder, alarm with radio)
Ensuring the management of the NLU activities
- Initiation and offering of a data analysis service to enable data-driven decision-making regarding the improvement and the expansion of NLU functionalities. The service was used by product owners and project managers to find out the root cause of problems, get deeper insights into the NLU and to be able to prioritize tasks. The service avoided losing time in exploring the data manually or not exploring the data at all;
- Initiation and implementation of an NLU KPI monitoring & analyzing the performance and the quality of the ML models on a weekly basis;
- Introduction and implementation of dashboards with automated daily updates of the performance of all NLU models (including the possibility to get performance metrics on-demand)
Benefits
- Our client successfully launched their Smart Speaker embedding a future-proof and expandable Virtual Assistant;
- Industrialized and optimized way of working on NLU activities (from working manually to automating the major part of the activities);
- Release of 15+ new features for customer use on the Virtual Assistant and improvement of the existing ones (including functionalities like smart home, radio, weather…);
- Improved decision-making and efficiency for the project managers and product owners with an NLU KPI monitoring and a “Data Analysis Service”;
- Comprehensive database with tens of thousands of labeled data sets for: developing, training and testing NLU models (in addition, this complete database can be used for other projects and is GDPR compliant by design);
At the end of the project, we were able to convince our client management to automate the NLU implementation and maintenance with a full NLU generation pipeline. The pipeline ensures the quality of NLU models and allows to scale the NLU implementation process to answer their additional need for creating several new models per week for B2B customers.
Teams involved on this project
Our team of 5 NLU/NLP Experts, 3 Data Scientists, 2 Linguists, and 1 Product Owner collaborated with our client on this project for almost 2.5 years.
Technologies and Partners
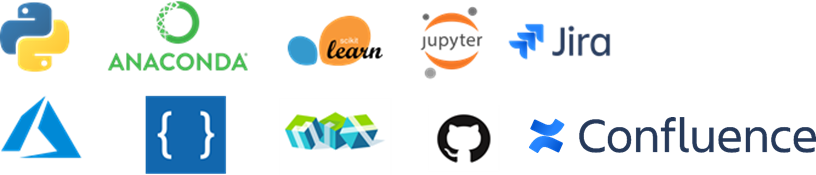
Python, Anaconda, Scikit Learn, Jupyter, Jira (Atlassian), Azure Cloud, Microsoft LUIS, Nuance Mix, GitLab and Confluence.
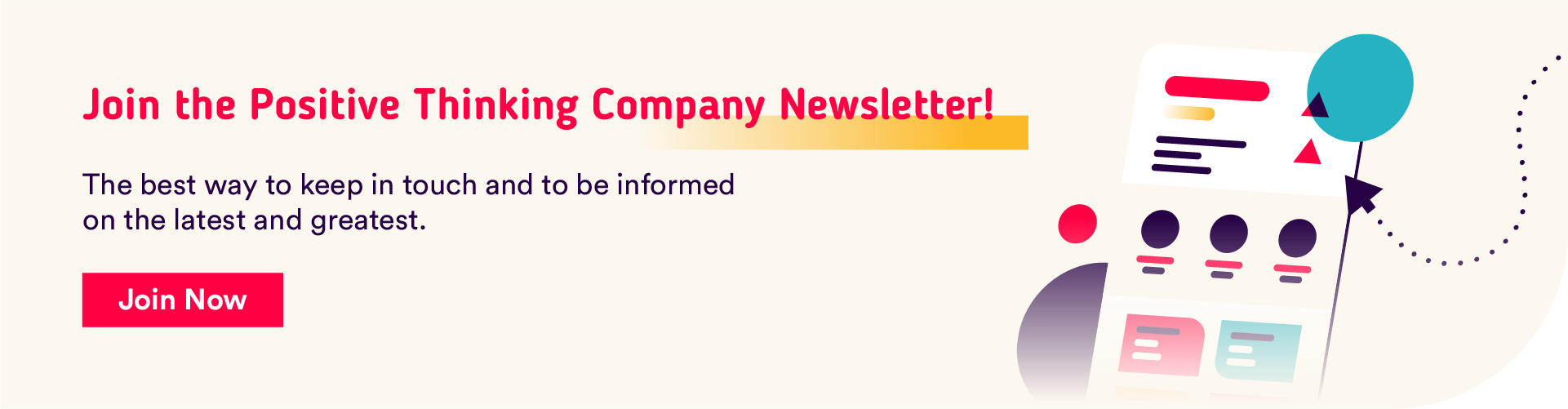