This case study explains how we supported our client throughout their AI journey from assessing their maturity to implementing a Data Science Platform, standardizing an agile project management and establishing data governance processes.
- AI Strategy
- AI Maturity Check
- Agile Project Management
- Model Deployment
- Model Development
- Machine Learning
- Data Governance
- Manufacturing
Key challenges
Our client is one of the leading German companies that produces white goods and that is recognized for the high quality of its entire product offering.
When we made contact with them, they were about to put their very first use case into production. They were about to move from the experimental phase to the operational phase of their AI journey.
In reality, our client had a very artisanal approach to managing their machine learning model lifecycle and a very decentralized data science organization and set of tools.
Therefore, they were looking for:
- Challenging their current approach and labs initiatives;
- Getting expert advice on how to structure and industrialize model development;
- Improving their go-to-market approach and the effectiveness of their data products.
Our approach
In order to address our client expectations, we:
- Performed our AI Maturity Check in order to accurately assess the current situation. We therefore analyzed the organization, infrastructure and processes dimensions in detail. Among others, we discovered that more than ten data science labs were in fact working on similar use cases, in ten different ways.
- Based on this comprehensive analysis, we provided a set of recommendations to enable our client to industrialize their initiative. Among them, we strongly insisted on the need to create a competence center including IT, Business and Data Science teams. Their role would be to manage a centralized Data Science Platform and provide guidance and processes for developing and deploying ML models.
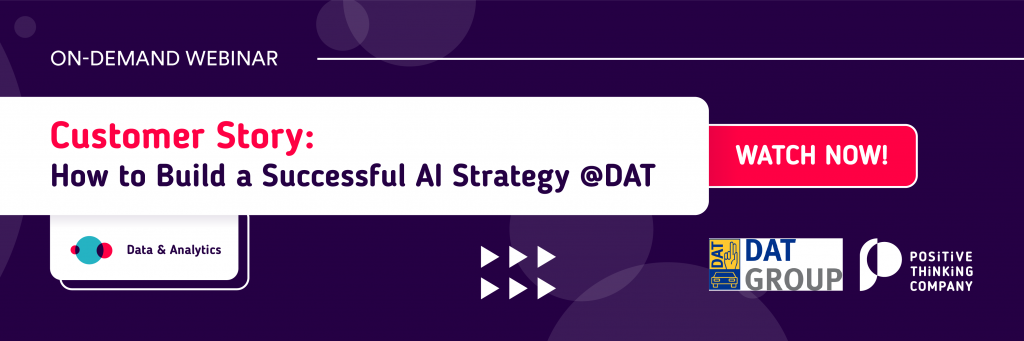
From that moment on and with a clear AI strategy in mind, our client decided to extend the scope of our intervention. We then supported them with:
- Creating the Data Science Platform in collaboration with the Center of Excellence and implementing MLOps best practices.
- Standardizing an agile project management structure and ensuring its adoption via Jira (from PoC to MVP to Production).
- Establishing the processes and governance of the data product lifecycle management.
- Implementing the first use cases to be deployed.
Benefits
- Clear and actionable AI Strategy with standardize processes and best practices.
- Increased efficiency while establishing innovative methods and becoming a so-called data-driven company.
- Centralized Data Science Platform, know-how and establishment of new roles (e.g. Data Architect), processes and governance rules.
- Proper use of agile project management frameworks with fully transparent project controlling.
- Audit-proof operationalization of machine learning models and data products.
Technologies & Partners
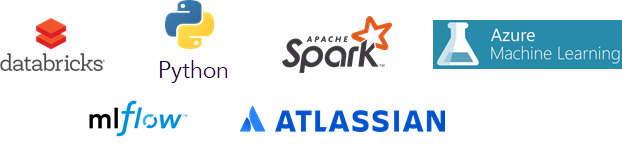
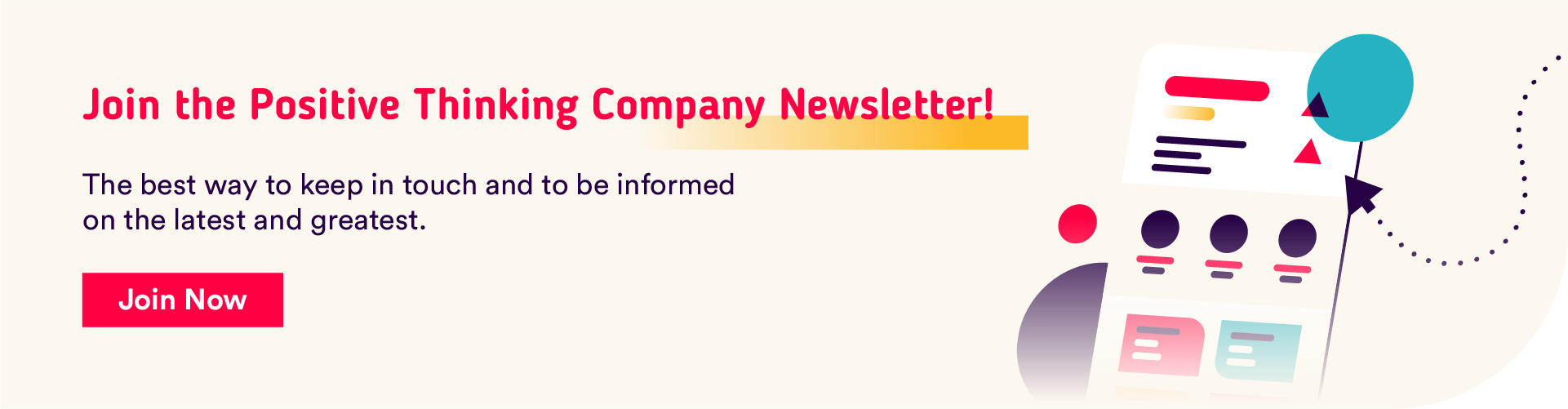