Nearly 3000 participants participated in the 11th edition of the Devoxx event.
This year’s main theme was artificial intelligence. But Java, Web, Cloud, DevOps, architecture and security were also on the agenda.
Several of our employees had the opportunity to attend the event, including one of them as speaker of the talk “Where is Data Science going?”.
Their expectations were varied:
Oscar: It was my very first Devoxx appearance. As a Data Engineer, I was particularly interested in the conference on ChatGPT, as well as the one on FoundationDB — This is a tool I discovered recently — and finally the multy-tenancy on Kafka. Not forgetting of course the conference of my colleague Daoud: “Where is Data Science going?”.
Frédéric: It was also my first participation at Devoxx France. My goal was to discover and learn about some technologies or practices related to devOps technologies, such as Kubernetes and Terraform. I am indeed making a professional transition from back developer to devOps.
Jeason: First participation for me too, and I had identified several interesting conferences before my visit.
I wanted to discover new technologies and deepen knowledge on front-end or web topics in general.
Thibaut: This is my first participation in Devoxx. As a back-end Java developer, I was interested to see the conferences around code best practices, Java and new technologies.
Data, Cloud, DevOps: 19 Devoxx 2023 Tech Conferences summarized for you
Frédéric, Jeason, Nicolas, Thibaut and Oscar share what they have learned from the main conferences they have attended.
The main theme of Devoxx was focused on AI, including the arrival of ChatGPT. Many of you have probably already tested it.
Two conferences focused on this topic:
Solve AdventOfCode with Github Copilot and OpenAI ChatGPTP
Is ChatGPT able to solve code problems with a statement? Well, yes and no! Because it can be wrong, but it can also question himself.
ChatGPT does not have the absolute truth and is even often wrong, but it can realize himself that he was wrong.
ChatGPT does not work at all on some issues, but it can be useful to save time by validating the code it generates.
Also, the composition GitHub Copilot and ChatGPT can be interesting.
ChatGPT Conversations: Illusion or Reality?
Why has ChatGPT been so popular?
An excellent conference to take a step back on the tool.
ChatGPT is far from the only pre-trained model available. But on the other hand, it is probably the first to be available for free and with a pleasant UX. This is undoubtedly the first reason for its success.
But even if it gives the impression of knowing everything, it is only designed to answer something plausible, not something true. That is why it can answer something incorrect very seriously. For example: Ask how to collect cow eggs. It will respond from what it knows, after learning many things, but does not check its sources. And above all it doesn’t know when it doesn’t know.
It also has a safeguard to filter sensitive topics, such as conspiracy theories, but this safeguard has its limits. It can be bypassed and presents a danger of use (e.g. it is possible to write functional malware).
Is it revolutionary in our professions? Well, not so much at the moment. We already have more and more AI in our tools (ex: GitHub Copilot). But ChatGPT is a little more powerful and simple. The tool is rather part of a technological evolution.
You should also know that the videos and images of the Devoxx were generated by AI. Impressive, isn’t it?
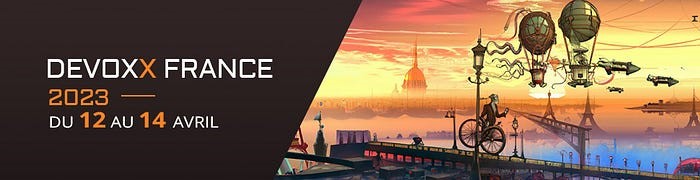
On other Data topics, we also find:
Where is Data Science going?
With the emergence of new terms within the data and devOps ecosystems, such as ‘Machine Learning Engineer’, it is appropriate to question the evolution of these professions.
Daoud, data scientist at Positive Thinking Company, analyzes and tries to provide an answer on the evolution of the Data Scientist profession.
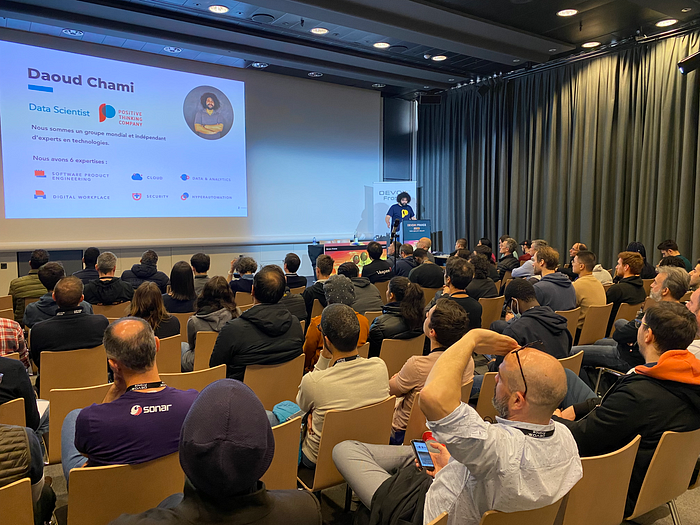
The role of the Data Scientist is, originally, to produce machine learning models. And therefore to have the business knowledge necessary for the development of feature engineering.
Data scientists then saw their profession defined by a Venn diagram, which placed the data scientist as someone who had skills in development, mathematics and statistics, and business expertise. Today, we see more and more diagrams that compare data professions with each other: Data Engineer, MLE, Data Scientist, Data Analyst. With a Data Scientist able to do (almost) everything.
However, it is still easy to explain the differences between the job of Data Scientist and Data Engineer or Data Analyst. On the other hand, the complexity is much higher when it comes to comparing it with the Machine Learning Engineer.
The Machine Learning Engineer’s main role is to automate machine learning models, and to deploy them.
This difference will be reflected in particular in job offers. Above all, the Data scientist is asked for business expertise skills, which is not at all the case for the Machine Learning Engineer, who is systematically asked for Ops expertise.
To conclude, the job of Data Scientist is probably not going to disappear in favor of Machine Learning Engineer. Which is ultimately a more devOps-oriented business but with specific expertise on the deployment of machine learning models. The Data Scientist, on the other hand, who was increasingly asked to know how to “do everything”, as for a full-stack developer, could potentially return to the basics of his job, which is to produce ML models.
In terms of tools and best practices, our employees have also retained these conferences:
Multi-tenancy at Apache Kafka, navigation in a major topic
Florent and François worked on a multi-tenancy solution with Apache Kafka. They detail, in a very playful way, the thought process they had to produce this solution.
Kafka is found almost everywhere. In the same organization, you can even find several Kafka instances, with several different customers, on several environments, in several teams, and for several different needs.
How to pool this? Can they be brought together under the same cluster?
Several solutions can be considered:
- Agreeing on a collective agreement
The major flaw of this solution is that it requires a social contract. - Provide a library
This can be very expensive and requires users to all familiarize themselves with this new solution. - Making “magic” between clients and Kafka cluster
This magic will be a gateway that will communicate with Kafka brokers. The gateway rewrites meta data, and makes assertion of messages. Multi-tenancy is now transparent, this gateway is accessible and responds in the same way as a kafka cluster, but adapts and changes its response according to the client.
This solution makes it possible to manage multi-tenancy, to add security, and therefore also, to use only one cluster per organization, and therefore to optimize costs. This gateway makes it possible to “rethink” Kafka according to his organization.
Storybook, a really good idea?
Storybook is a tool for designers and developers.
It makes it possible to provide a design system and avoid the multiplication of the different components of a site. It also avoids the side effects of modifying existing components. Having a consistency of buttons or other components, also allows a better user experience.
Storybook still imposes some constraints: It requires regular maintenance to remain useful and not to weigh down and confuse the code.
But to quote Sara Attallah: “StoryBook: To try is to adopt it.”
Revisiting Design Patterns after 20
A design pattern is a reusable solution, for a recurring problem, in a given context.
Its design principles are:
- Composition is preferable to inheritance because it is more flexible.
- Small interfaces are preferable to large interfaces, as they are more stable.
- Interface reuse is preferable to interface creation.
Before jdk20, several classes and/or interfaces were required to implement a design pattern. Thanks to the functional approach, it is now possible to assign fewer interfaces, and even in some cases a single interface, to a design pattern.
Implementation then becomes easier and more maintainable. Functional programming has significantly simplified the implementation of design patterns in Java 20.
Java 19 & 20 : What’s new and noteworthy ?
In addition to simplifying the implementation of design patterns, find the main highlights of Java 19 and Java 20:
- Just because a version of Java isn’t LTS doesn’t mean it shouldn’t be used.
- The most striking novelty, since lambdas and streams in Java 8, concerns the management of “virtual” threads.
- Other important new features are to be noted, such as pattern matching, record matching, native code…
Secrets in the pixels! Discovering steganography
How can images hide secret data in their encoding?
- A PNG image contains several sections. It is possible to add information after the end of the image, or even of scripts.
- Two separate base-64 encodings can represent the same text. This is due to the grouping of bits in groups of 6. It is therefore possible to hide information in a base-64 string.
- It is also possible to hide information in the pixels of an image by relying on RGB encoding.
Let’s give DDD back to developpers
How to refactor a code that is difficult to read and roughly maintained through small steps, and then focus on design in order to decouple the domain from the technical part?
- Before refactoring, check test coverage. It ensures that refactoring does not lead to regression.
- During refactoring, with each modification, even minor, replay the tests and commit. This ensures that nothing is broken and that you can go back if not.
- Declare and initialize variables as close as possible to their use
- Use the “sandwich” pattern so that the technical and business parts are not intertwined.
- Apply hexagonal architecture in order to free the domain from technical dependencies.
Container Builders : Which is the best image builder ?
For this conference, a maze generation application was developed. It is containerized with several different tools.
The goal is, as a first step, to compare the size of each image produced. In a second step, it is a question of comparing the startup time, the throughput and the latency of the application.
The technical environment is: Java 17, Spring boot 3, compliant OCI docker image.
In comparison with the sizes of the different images produced :
- Docker + Jdk : 380 mb / Docker + Jre : 190 mb / Docker + Custom Jre : 113 mb
- Jib : 289 Mb
- Cnb : 278 mb
- GraalVM : 98 mb
Comparison to boot times, throughput, latency and image size: JIT compilation based solutions (Docker + jdk, Docker + Jre, Docker + custom Jre, Jib) have better throughput and latency.
AOT compilation based solutions (GraalVM, Spring3 + GraalVM) have a smaller size and shorter boot time.
In the end, there is no magic solution, everything is a question of the environment, constraint and compromise.
Alice in the land of OpenTelemetry
OpenTelemetry refers at the same time to a data standard, a resource naming convention and the implementation of optional application instrumentation.
OpenTelemetry solves the absence or lack of observability for an application and refines observability, covering all layers of an application.
It allows this thanks to the definition of collectors. A collector is composed of several pipelines. A pipeline is composed of a chain of receivers, processes and exports.
To apply it, some good practices are to be remembered:
- In terms of metrics, OpenTelemetry is not a back-end data. It is therefore necessary to think about defining its back-end data (Prometheus or Grafana for example). For a better correlation, it is also necessary to annotate its resources. For this, it is possible to use the K8s Attributes processor that automatically annotates your resources.
For more flexibility, you can also use sidecars that act as intermediaries between your resources and the collector. Receivers are interoperable. They implement HTTP and gRPC protocols. - As for logs, they are sent to collectors by daemon sets installed in the cluster. So for more security, you must manage the privileges of your pods in the cluster. For more performance you can also use special operations in receivers to include/exclude/recombine/filter logs.
You can also use special processors such as search and replace. - Finally, correlation is not managed natively. Customized configurations are therefore to be expected.
CRAC VM vs GRaal VM: For a quick start
Java application deployment has evolved over time with: Java archives, application servers, microservices, embedded servers, and serverless functions.
The goal of the JVM remains, among other things, to improve the performance of an application in general, and its start and query execution time in particular. The JVM uses JIT profiling compiler to achieve this.
But some operations are greedy, such as loading classes, managing annotations, initializing static blocks and initializing the application complex (Container Sping, CDI, etc.).
For this, we find the GRAAL VM and CRAC VM solutions. CRAC VM is incubated by OpenJDK while Graal VM is incubated by OracleLabs.
Graal VM uses the AOT compiler. The latter compiles the bytecode into native code to speed up the startup of the application. However, this solution has some disadvantages, such as:
- Reflexivity is lost at runtime.
- The JVM is replaced by the VM substrate, which is less efficient than the first.
- The Garbage collector is replaced by the Serial GC, less efficient than the first.
- Dynamic optimization is replaced by static optimization, which is less efficient than the former.
For example, during the demo, a simple ‘helloworld’ took 5mn28 build time. It started in 0.038 s.
Crac VM uses a checkpoint/restore system. It saves the JVM in an optimal state and restores it later. The ‘jcmd XX checkpointTo’ and ‘XX restoreFrom’ options provide restore and backup operations.
For example, during the demo, a prime number checking application was saved and then restored to 0 ms (almost zero startup time).
Bottom Line: CRAC VM is preferable to GRAALVM because it reuses the native components of the JDK and therefore has better compatibility.
Cloud Native Security for the rest of us
Find a list of best practices and recommendations to apply with Cloud Native Security :
- Secure traffic to your cluster, for example with TLS checker.
- Protect your nodes from your pods by controlling and configuring appropriate security contexts.
- Update your cluster regularly.
- Isolate your virtual networks using ingress and network policies, and service mesh and cillium for more complex network policies.
- Protect your sensitive data in an external Vault, such as HashiCorp Vault, Sealed Secrets.
- Secure your communications by applying Encryption at Rest.
- Define the appropriate authentication method: Human Token, Robot Service Account, etc.
- Define the appropriate access control policy: Role Base Access Control, Attribute Bases Access Controls, Webhook, etc.
- Apply DevSecOps by protecting your supply chain: Sign your assets with Sigstore, scan and correct CVEs, control compliance with Open Policy Agent, …
- Religiously apply OWASPs.
- Set up a solid observability of your cluster and deployments with Prometheus and Grafana for example.
FoundationDB: The best-kept secret of new distributed architectures!
FoundationDB is an open-source database. It can, on its own, solve many problems encountered when working on this kind of tool.
Too many databases have different uses. The first question to ask is: Can we mutualize?
FoundationDB is not concretely a database, but simply a base that allows this mutualization. This foundation consists mainly of a transactional storage engine. It is then possible to produce layers on this engine according to the uses.
With an Actor model architecture, each problem is represented by actors. This makes the architecture more scalable. It is then enough to increase or decrease the number of actors on a type of task to optimize its performance and cost. This solves most tuning problems.
Only defect, the tool keeps in memory 5 seconds of mutation (what we call resolver). The maximum time of a transaction is therefore also 5 seconds.
SQL (the return): Let’s demystify preconceptions and use DB effectively
Devoxx offered other conferences on relational databases. These databases are not forgotten, but less and less used with the emergence of NoSQL databases and their rather aggressive advertising. Nevertheless, SQL databases are probably more promising in the future, because they are and remain very powerful. They can meet multiple needs, which we would not necessarily have thought of, as this conference demonstrates.
30 indexes on a 6TB PG table: Challenges and solutions
Another conference, also on SQL, was presented by Doctolib. It was a feedback on the problem: How to reduce and optimize a postgreSQL table of more than 5TB?
With solutions: Deletion of unused indexes, merge of indexes and creation of partial indexes, and development and provision of an open source tool (transaction-based) to receive this update.
From Chroot to Docker, Podman, and now Wasm modules, 40 years of evolution of containerization
A bit of history also with containerization, which began more than 40 years ago.
We offer you some (non-exhaustive) dates to remember:
- 1970 — Birth of containers in Linux systems, with their fundamental problems: Sharing limited resources and separating production and development.
- 2006 — Process container (cgroups): Sharing based on limitation, quantification and isolation. This is the basis of modern insulation.
- 2011 — Warden : Automates the management of LXC containers thanks to an API.
- 2013 — Docker : Reuse of user namespaces, replacement of LXC by libcontainers and provision of an API to automate the creation of containers.
- 2016 — WebAssembly (WASM): Sharing based on the execution of bytecode in an isolated sandbox. This allows for very good portability, performance and security.
- 2022 — Docker supports Webassembly.
Remember: WASM is the most successful container runtime to date, so it is supported by Docker.
Not to mention related topics, but just as interesting, during kick-offs in particular, including:
Journey to the center of the watch: Continuous learning with your technological watch
Less technical conference, the subject was focused on how to do monitoring, with several tips for an effective and organized watch.
There are two types of technological watches:
- We talk about professional monitoring when it is the company that gives time dedicated to it. This is therefore often limited to the proposed framework.
- When personal monitoring is on the personal time of the developer, and therefore on the topics and formats he wants.
It is especially on this type of monitoring that the following tips are applicable:
- First you have to source. It is important to organize your sources and keep in mind that each source is equivalent to a “task” that must therefore be patiently organized as such, in order to avoid a risk of accumulation.
- Then, we must treat these sources, and therefore consume them. But it is also necessary to take notes, which helps working memory, memorization and feed a reflection. These notes must be filed and reworked, to avoid keeping notes that will never be proofread.
- We can finally value this monitoring, by sharing our knowledge and innovating from it (linking ideas together to produce things).
The conference concludes with some tips to start your watch.
It is specified to define its monitoring objectives and to define its own process. But also, to really find and take time for it, while keeping the idea of finding your own rhythm. And if you use tools, start light and then adapt them to your needs.
Digital is for everyone… Or not!
This very interesting conference addresses the topic of inclusive web.
Inclusivity is the way to address the issues of non-access to the digital world by users, and makes it possible to realize certain aberrances that we encounter during the development of tools.
The subject includes everything that is an obstacle to access to digital tools, of which here is a non-exhaustive list:
- Accessibility on the web.
- The weight of sites, driven by the development of new tools: Not everyone has access to the latest iPhone or 5G.
- Mobile network coverage in white areas.
- The digitization of tools that have no physical alternative.
- …
The speakers concluded by encouraging people to learn about these topics that are still too little discussed.
“40% of French people are worried about carrying out procedures online and 34% of residents of medium-sized cities say they do not take advantage of the opportunities offered by digital technology at all. ” — Digital society.
Varied Tech conferences and a Devoxx 2023 that lives up to expectations
The conferences met the expectations of our employees and allowed them to deepen many topics on various expertise.
Oscar: I am very satisfied with this first participation. Each of the conferences taught me things. With in addition some very nice meetings on the stands! And congratulations to Daoud for his presentation, solid to the end!
Frédéric: I am very happy to have been able to attend this great event. I come out with a lot of informations that I will be able to put in place in my personal or professional projects. I was also able to discover other topics that I had not initially planned, but which are just as interesting.
Jeason: I loved the day and learned a lot. But for next time I might focus on a conference for front-end developers.
Thibaut: This first Devoxx experience was really enriching! I really enjoyed being able to pick from varied and interesting topics.
We regularly publish articles on topics of web and mobile product development, data and analytics, security, cloud, hyperautomation and digital workplace. Follow us on Medium to be notified of the next articles and carry out your professional watch.
You can also find our publications and news via our newsletter, as well as our various social networks: LinkedIn, Twitter, Youtube, Twitch and Instagram.
Want to know more? Check out more of our website and our job offers.