AI has grown up. Over the last years, many organizations have successfully experimented with AI and, as a consequence, are now moving towards operationalizing models and capturing the resulting business value.
Why should you care about MLOps?
Unfortunately, companies are struggling on their path to fully operationalized AI: According to a study from Algorithmia Research, 38% of Data Science teams now spend more than half of their time on deploying ML Models and 83% of Data Science teams spend at least a quarter of their time on ML Model deployment.
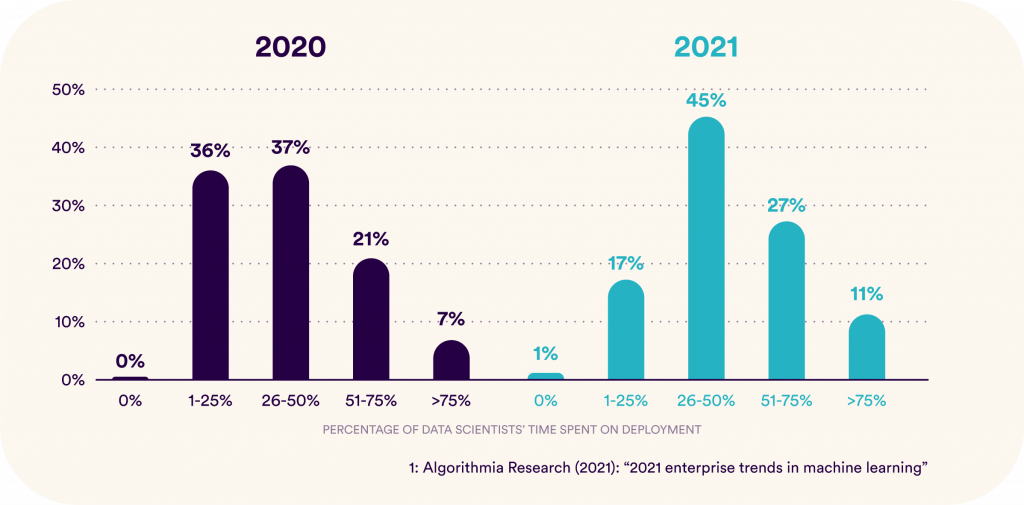
This circumstance carries significant implications.
From a high-level perspective, successful AI use cases can be split into two parts.
- Creating value by building models
This is the phase where you train your AI to handle business tasks and process steps which would have been deemed impossible to be accomplished by a machine only a few years ago. This creates new, previously unattainable business value. - Capturing value by bringing these models into production
Once such an AI has been trained, it needs to be integrated into the existing technical infrastructure and organizational processes in order to reap the benefits of the newly found capability. The value potential created during the first step is captured during this phase.
If you want to get the most out of AI for your business, you want to spend as much time as possible on the first phase – which creates new value – and only the minimum amount necessary to capture the value on the second phase.
Unfortunately, the data above shows that for 28% of companies, reality is quite the opposite.
They spend more than 50% of their time on capturing value, also known as deployment, instead of creating new value.
This is especially critical as this trend is worsening. The percentage of teams that spend more than 25%, 50% or even 75% of their time on deployment is increasing on a year-to-year basis.
Once we dive deeper into the numbers, we can draw another conclusion: The time organizations spend on ML model deployment increases with the number of models in production.
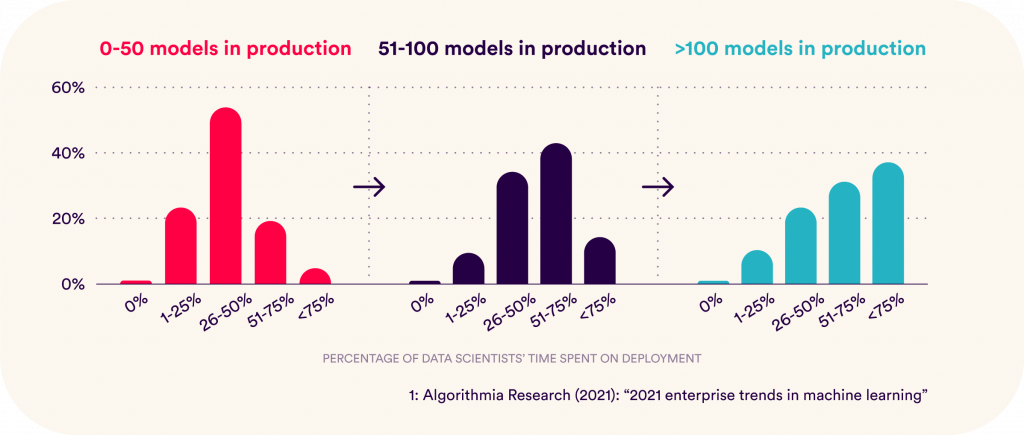
We can summarize those findings: At the moment, Data Science teams do not scale very well.
And from our experience, the reason is pretty clear. Without a robust framework in place, the work related to deploying and operating ML models increases linearly with the number of models in production. And we must keep in mind that once a model has been deployed, it needs to be monitored, updated and potentially retrained on a regular basis. While the actual deployment might be a one-time effort, each model introduces an additional amount of recurring work for your team. If no precautions are taken, the team will reach a point where it spends all of its time on monitoring, maintaining and retraining existing models.
So, what are the factors that drive this relationship and make it so difficult to deploy AI use cases at scale?
Again according to Algorithmia Research, there is a multitude of reasons.
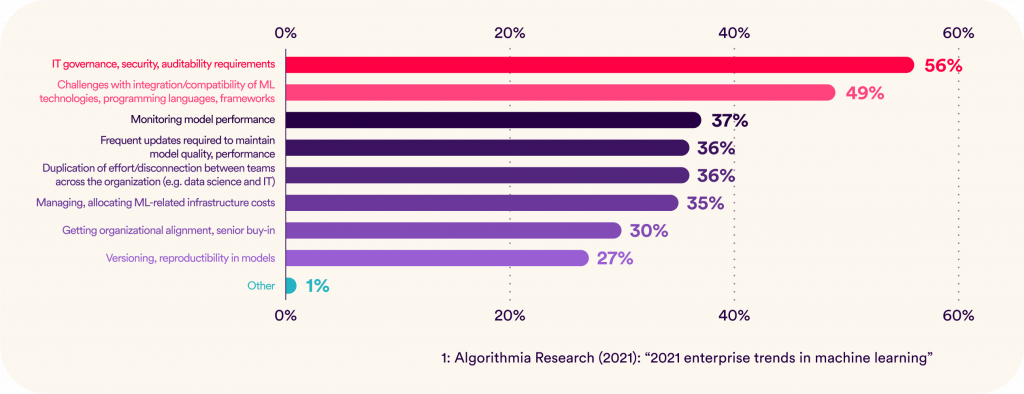
The data shows us a number of challenges ranging from IT governance, security and auditability to versioning and reproducibility. It also tells us that only 1% of companies struggles with other, additional issues. The points stated in the graphic above are, by far, the most dominant ones. Therefore, if you want to steer your data science teams towards scalability, those are the challenges we advise you to tackle first and foremost.
This gives rise to our main question: How can my company take action and avoid falling into this deployment trap?
There is a five-letter answer to this question: MLOps.
How can you approach MLOps the right way?
Formally, MLOps “focusses on streamlining the process of taking machine learning models to production, and then maintaining and monitoring them”. –Databricks.
In practice, it is about building a platform that allows your data teams to scale.
We suggest you look at your future MLOps platform from three perspectives.
1. Design
Start by designing your MLOps Platform according to the individual needs of your company.
About one third of companies struggling with model deployment reports that they have trouble with duplicated efforts, disconnection between teams across the organization and getting organizational alignment as well as senior buy-in.
By designing a centralized, organization-wide platform, you avoid duplication of processes, work and efforts. You connect and enable teams to collaborate through your all-in-one platform. By integrating the need of every (senior) stakeholder, you create organizational alignment and buy-in.
2. Operation
Once a suitable MLOps platform has been designed, it needs to be built and operated.
But be aware: This is where the next major pitfalls hide! Every second company has difficulties integrating all the different ML frameworks, programming languages and tools that are out there. At the moment, there is no single, one-size-fits-all MLOps solution available. In consequence, to actually implement a platform that is truly tailored to your needs, combining different tools and technologies is a must. And therefore, picking and combining the right tools that work together smoothly and achieve the wished-for capabilities within your platform becomes critical.
A well-implemented platform is secure and auditable. Every asset, from data to code and trained models, is versioned and reproducible. Production logs and metrics are stored in a central location and model quality is constantly monitored. And frequent retraining does not prevent your Data Science team from building new models because of rigorous automation. Every phase of your Data Science process is highly automated: From data extraction, preparation and preprocessing to feature engineering and model training to deployment and instrumentation. This enables your team to focus on the work that drives progress by freeing them from any tedious recurring tasks and time-consuming housekeeping. It allows your team to scale.
3. Organization & Processes
Even the best platform cannot create value if it is not utilized properly by its stakeholders.
In consequence, you will face the challenge of integrating your new platform into your existing processes and organizational structures. This gives rise to a considerable number of questions.
- How should Data Scientists and Engineers work together to fully reap the benefits of the platform?
- How do you make sure that confidential data is dealt with appropriately?
- How do you assure data and model quality?
- At which point do models have to be approved?
- And by whom?
- What must be documented?
- Who has access to which areas of the platform?
These are just some examples of the plethora of questions you will have to answer. It is no coincidence that governance ranks number one in the challenges listed above and 56% of companies struggle with this task.
But there is also a bright side. Once you have managed to set up the right organizational structure and processes, your data starts flowing smoothly through the platform: From ingestion to analysis, training, evaluation, approval, deployment and finally operation.
The time from raw data to working product is minimized and your team is able to focus on building new models instead of maintaining existing ones.
They maximize their time spent on creating new value and spend only the minimum time required on capturing value.
Don’t want to walk this path on your own?
These steps are challenging hurdles to take.
At Positive Thinking Company, we speak from experience. We have consulted a variety of clients from different industries on all maturity levels during each of those steps multiple times.
During this time, we have developed a number of best practice designs that can be tailored to your needs. We have built and operated a large number of those designs by recommending tools and frameworks and making them work together. And we have advised our clients on the necessary adjustments to their processes and organizational design.
Below are some examples of projects involving MLOps expertise on which we have collaborated:
- Building a Platform to Optimize Customer Service Request Process through Microservices, in utilities
- Deployment of a Biometrical Risk Prediction Model for a public insurance
- Effective Organizational Embedding of Data Science & AI for a leading manufacturing company
- AI Strategy and Implementation for Deutsche Automobil Treuhand (DAT)
If you would like to know more on how we achieved this and how we accompany our clients on their way to utilizing data and AI at scale, get in touch with our teams now
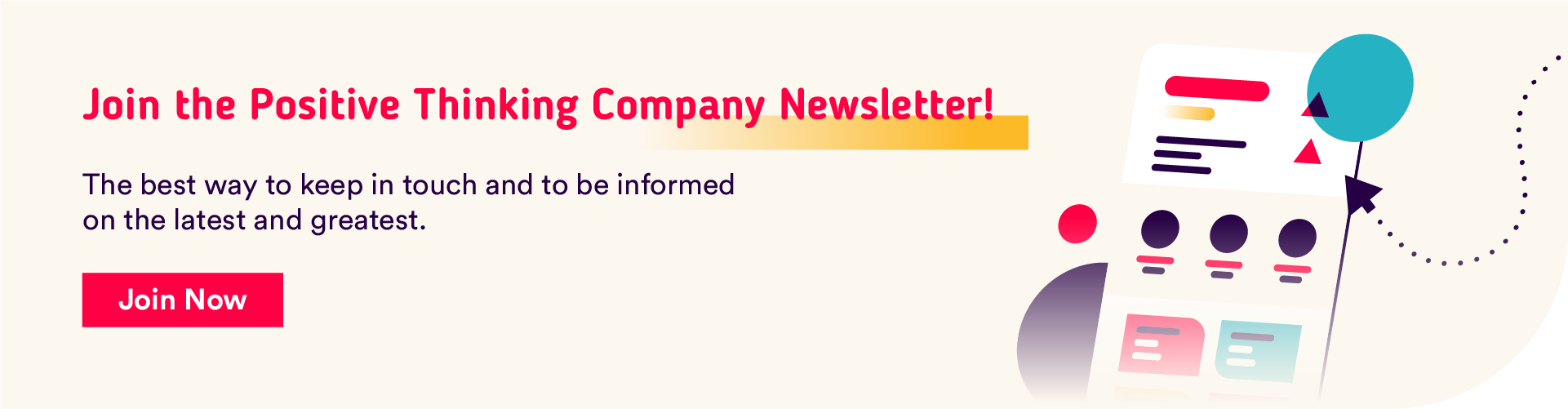