Let’s envision for a moment a future with robust and reliable Large Language Models (LLMs) with fewer weights, and fast inference times, which are production-friendly and thoroughly evaluated.
The path towards achieving this goal is not to gather larger and larger datasets and train LLMs with even more model parameters. Interestingly, the opposite “Less is more” approach is much more promising. Data-centric NLP techniques generate high-quality, debiased, information-rich datasets for pre-training, finetuning, and alignment in a semi-automated manner. The challenging discipline of Data-Centric NLP focuses on developing datasets at scale and gives model optimization a secondary priority.
But what makes this approach pivotal? Why should Data Science and AI teams even consider it?
This article aims to demystify the concept of Data-centric AI, elucidating why it’s making a substantial impact in Generative AI and NLP, and how you can practically implement it in your projects. Through a clear and comprehensive exploration, we’ll dive into the essence of Data-centric AI, providing valuable insights to both business and technical audiences.
Content
What is Data-centric AI?
Data-centric AI is a straightforward yet powerful concept: the quality and expressiveness of your data is central to the success of AI projects. Let’s break this down into simpler terms.
Data-centric AI is the discipline of systematically engineering the data used to build an AI system.
— Andrew Ng
For more: have a look at “A Chat with Andrew on MLOps: From Model-centric to Data-centric AI DeepLearningAI” video on Youtube.
In traditional AI projects, a lot of focus is put on the models and algorithms. Think of the model as the engine of a car. A lot of effort goes into making this engine as powerful and efficient as possible.
However, in a data-centric approach, the focus shifts towards the data, which you can think of as the fuel for the engine. Just as a car runs better with high-quality fuel, an AI model performs better with high-quality data.
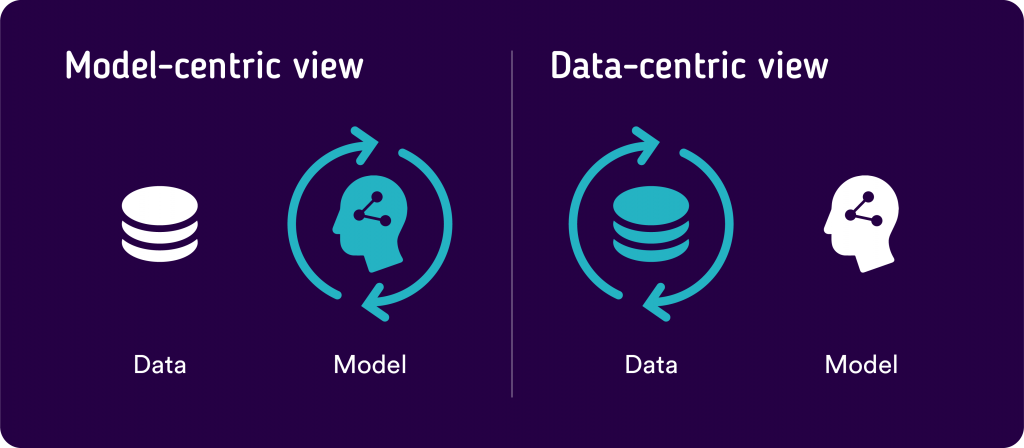
This means that instead of spending most of our effort on tweaking the engine, we ensure that we’re using the best fuel available. In practical terms, this involves:
- cleaning up the data,
- making sure it’s relevant,
- and that it accurately represents the problem we want the model to solve.
For both business and data experts, this approach emphasizes the importance of the data you feed into your AI models. Better data leads to better performance, making your AI projects and products more successful and reliable.
Why Data-centric AI is a Game-Changer in NLP?
Well, why is focusing on data such a big deal in NLP? Below we explore why this approach is making waves and changing how we handle Generative AI and NLP projects.
- Improving Model Performance: When the data is clean and representitive, AI models can learn generic patterns more effectively. In NLP, this means that models can understand and process language in a way that’s more accurate and useful.
- Saving Time and Resources: By putting an emphasis on quality data, we can save a lot of time that would otherwise be spent constantly tweaking and adjusting the AI models. This makes the development process way more efficient. Just consider how hard it is to perform regression test for LLMs upon redeployment, to ensure a new model version is at least as good as the previous model version.
- Enhancing Adaptability: With a strong foundation of quality data, NLP models become more adaptable. They can better handle new information and changes, making them more versatile and reliable in real-world applications. Consider the dataset as a kind of schoolbook. If the key concepts are clearly outlined, it is possible to “connect the dots” and come up with inspiring new ideas. If irrelevant and duplicated content is shown to a learner, it is hard to develop a useful mental model of knowledge to be recombined.
- Facilitating Better Decision-Making: In business, having an NLP model that provides accurate and reliable results means that decision-makers have better information at their fingertips, leading to more informed and effective decisions.
In simple terms, a Data-centric approach makes any NLP project more robust, efficient, adaptable – which is essential for meeting the diverse and dynamic demands of the rising LLMs.
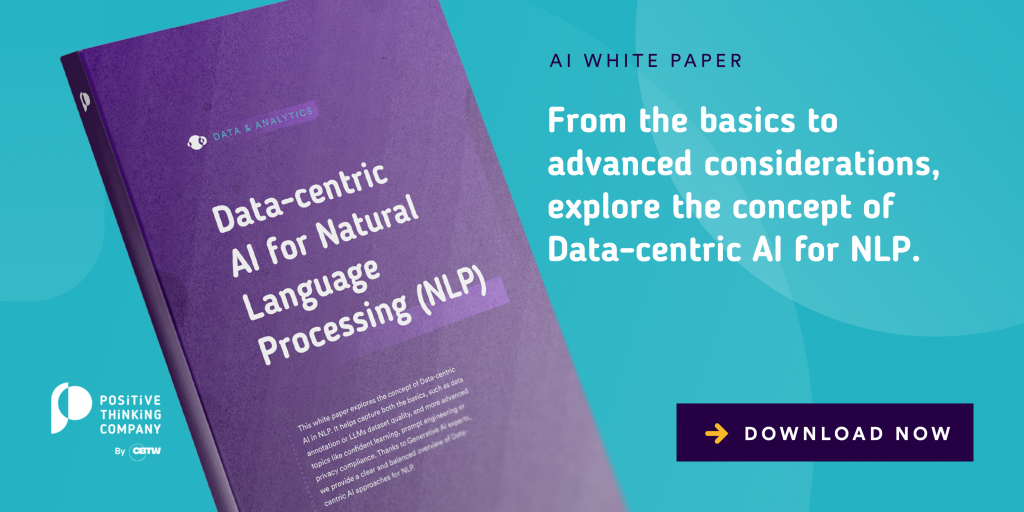
How to Implement a Data-centric AI Approach?
Implementing a Data-centric approach in your NLP and Generative AI projects at scale (for terabytes of text data) might seem daunting, but it doesn’t have to be. Here are some practical steps and insights to guide you through the process.
1. Data Collection and Organization
- Focus on quality: Try to collect high-quality and relevant data from the first place. Ensure that the data is representative of the real-world scenarios you want your model to handle. It will pay out in the long run.
- Pay attention to organization: Organize the data in a structured manner, making it easier to access and use during the model training process.
2. Data Cleaning
- Remove irrelevant data: Not all data collected will be useful. Identify and remove information that doesn’t contribute to the model’s learning process.
- Handle missing data: Decide how to handle gaps in the data, whether by removing such instances or filling them in a logical manner.
3. Data Annotation
- Adopt a labeling strategy: If your project requires labeled data, ensure that the labeling is accurate and consistent.
- Utilize tools and services: Consider using available tools and services that can assist in the data annotation process, making it more efficient.
4. Continuous Improvement
- Implement feedback loops: Implement feedback mechanisms to continuously improve and refine your data and models.
- Stay updated: Data needs can change over time. Stay updated on the latest trends and best practices in Data-centric AI to keep improving your processes.
Adopting a Data-centric approach is about focusing on the data as a key component in the success of your AI projects and products. With quality data, and the right approach, you can improve the performance and reliability of your AI models, ensuring the success of your projects.
What are the Future Perspectives on Data-centric AI in Generative AI and NLP?
Looking forward, the emphasis on Data-centric AI is poised to continue shaping the trajectory of Generative AI, NLP, and LLMs innovations and applications. Here’s a glimpse into what the future might hold as this approach becomes more deeply integrated into AI projects.
1. Enhanced Model Performance & Lower-Inference Latency
As more projects adopt a Data-centric approach, we can expect to see improvements in how NLP models and LLMs perform, making them more accurate and effective in various applications. Smaller models might outperform larger models and result in low inference latency, better maintainability, and lower deployment costs.
2. Broader Applications
With improved data quality, NLP models could be applied in more diverse areas, expanding their usefulness and impact across different industries and sectors.
3. Improved Collaboration
A focus on data can facilitate better collaboration between technical and non-technical stakeholders, as it allows for a clearer understanding and alignment of project objectives and outcomes.
4. Ethical and Responsible AI
A data-centric approach promotes the consideration of ethical implications, encouraging the development of NLP models that are more responsible and sensitive to societal impacts. Regulatory compliance and AI safety is guaranteed if compliance already takes place at the level of the training dataset. This might reduce the effort of content moderation filtering after model deployment.
5. Continuous Learning and Adaptability
Emphasizing data can lead to models that are better at learning and adapting over time, making them more resilient and capable of handling new challenges and changes in the language.
In other words: the road ahead for Data-centric AI in NLP looks promising, with the potential for numerous advancements and improvements that will drive success in various projects and applications. It’s an evolving journey that carries the promise of making Generative AI, NLP, and LLMs more robust, adaptable, and aligned with real-world, business needs and challenges.
Data-centric AI – Takeaways
Navigating the complexities of Generative AI and NLP can be a very challenging journey. However, adopting a data-centric approach has the potential to be a transformative strategy that improves the performance, adaptability, and success of your AI projects. You’re now equipped with a foundational understanding and actionable guidance on the key concepts, practical insights, and future perspectives of data-centric AI.
As we stand on the threshold of new possibilities and innovations in NLP, embracing data-centric practices presents a compelling opportunity to drive progress and achieve remarkable outcomes. For a more profound exploration and comprehensive insights into maximizing the potential of Data-centric AI in Generative AI and NLP, we invite you to download our complete white paper on the topic.
More Resources on Generative AI and NLP
Explore other content and tutorials from our recent LLM Mini-Series:
- Episode 1 – API Integrations with LLMs: Talking to the Databricks API with text-davinci-003
- Episode 2 – Parallel Multi-Document Question Answering With Llama Index and Retrieval Augmented Generation
- Episode 3 – From Unstructured Data to Structured Data with Open-Source LLMs
- Episode 4 – Querying SQL Databases in Natural Language Using the Langchain Library
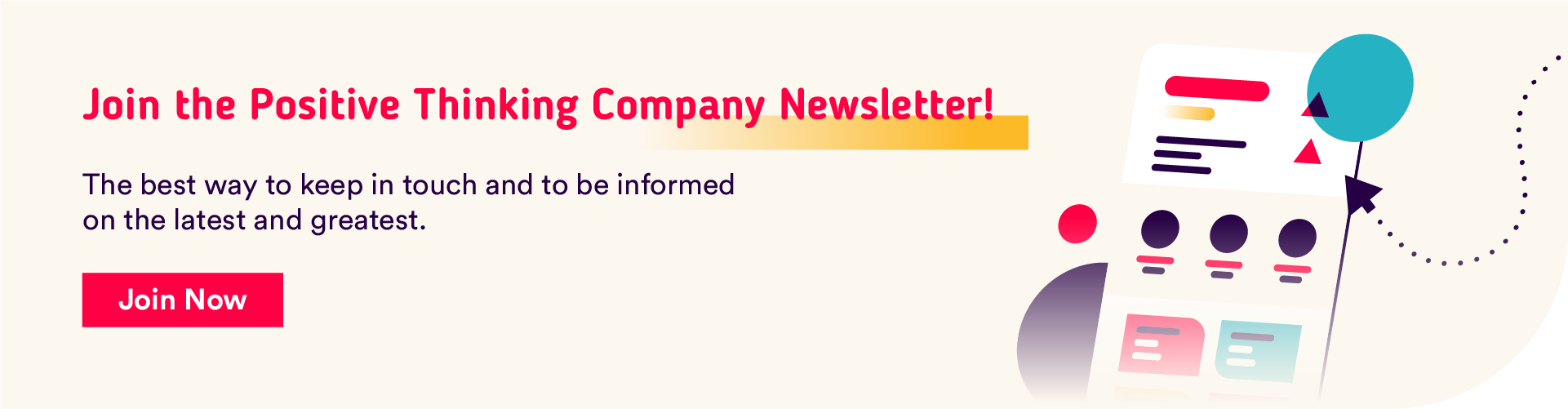