You have deployed self-service analytics tools, such as Power BI or Tableau. Your goal was to get your business teams to become data-driven. Yet, a few months or even years later, the expected benefits are not (all) there and you may find that:
- The adoption of such tools is quite low, although you rolled out a dedicated training program.
- There is a proliferation of reports, with various versions of the same report on the production server, or a lack of consistency within your reporting strategy.
- Scaling local initiatives is difficult and cross-domain analysis is still tedious and time-consuming.
How could you address some of the above issues? In this article, you will find the four key areas you should act on. You will learn more about root causes and best practices to unlock the full analytical potential of business teams.
Data literacy: Do you speak data?
When you deploy self-service analytics tools, you provide tools to business teams that are more user-friendly than legacy Business Intelligence (BI) tools. Yet, their user-friendliness does not eliminate the need for data literacy – understanding data and how to use it to solve business problems1.
The members of your BI team not only know how to use their BI tools, but they also have a background in data preparation, data modelling, and data analytics: unlike most of your business teams, your BI team is “fluent in data”. You may argue that business teams should have a lower data literacy than your BI Team. It is correct, but also implies that a minimum data literacy is still required for your business users (and, by extension, all your report and dashboard / BI consumers).
If you want your company to become (more) data-driven, you will have to make sure that your co-workers “speak data”. They require a broader understanding of data, beyond the simple but limited scope of learning how to use a self-service analytics tool.
We advise you to assess data literacy within your staff and plan specific training sessions for those who need to develop it. Such training includes topics like reading and interpreting visual data, data ethics, data preparation, analytics, and communicating with data. Although the topics are generic, make sure that you illustrate your points with real-world examples and, if possible, from the domain of your business teams. It will make it easier for them to relate to these use cases and assimilate knowledge.
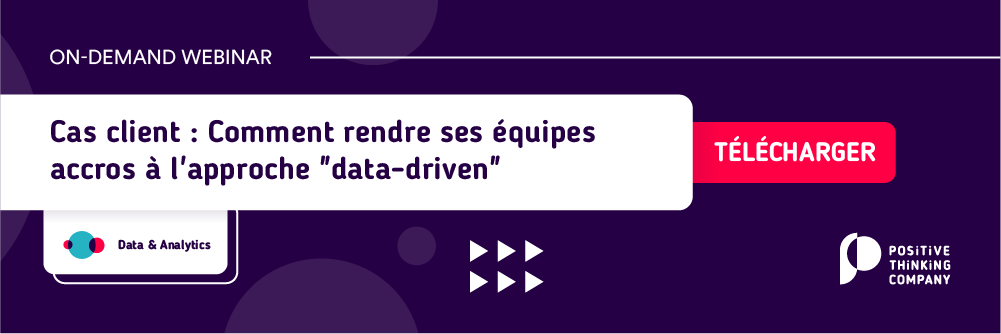
A change of paradigm leading to a shift in roles: The need for change management
Let’s face it: Deploying self-service analytics is not only about technology or even data literacy. It is a change of paradigm: now your business teams have access to data in a more autonomous way, which implies a new way of working. As humans, our initial reaction to change is usually to expect things to stay the same, to hesitate to change, to be reluctant to change, or simply to resist.
Being aware that these natural behaviours might impact the adoption of a self-service analytics approach is the first step. The second one is to realize that successful change management goes beyond communication and training alone. How many IT projects do we know failed because they did not consider thought-through change management, or if they did, it was only communication and training at the end of the project? Change management is a process that needs to be part of the full scope of any IT project from the start to the end.
To successfully lead change management, we advise you to work on the following pillars: Strategy, Sponsorship, Support, and Ownership. Activities should include defining your vision and involving key stakeholders right from the beginning, as well as identifying your sponsors and change ambassadors. Having team members who can speak both data and business is key, as well as being able to deliver intermediate results (not only at the end of a project) is critical to accelerate and ensure the project buy-in.
The need to establish solid foundations for self-service analytics
Additional analytics tools lead to additional requests being made on your Database Management Systems, not to mention the security and confidentiality aspects.This additional load can in turn lead to degraded performances. Slow response time might discourage your business users. As a result, a poor performance might also slow down the adoption rate of your new analytics approach.
On top of providing rapid access to data to your business users, you must also ensure the quality of this data:
- No data will always be preferable than bad data. Bad data are liabilities that can adversely affect your business. While no data are liabilities that can be managed (adding data sources for instance).
- You should always define and implement data quality rules to prevent erroneous data from reaching your end users. Even small inconsistencies in the treated data can create mistrust from your users, who will then abstain from further using your self-service analytics tools.
Implementing a self-service analytics approach is a major change for your company, and your organization should reflect this change. Your BI team should have previously worked quite closely with your business teams. However, requests from users recently converted to self-service analytics might overwhelm the BI team. On the contrary, the BI team might face a lack of responsiveness on business requirements. Both situations can hinder the deployment of self-service analytics.
To build solid foundations for self-service analytics, data should be trusted, and its access made swift, easy, and documented within your organisation. You can take advantage of faster in-memory databases. Structure and roles in your company must evolve, if needed, to account for the requirements brought by the democratization of data.
We recommend appointing data stewards and making every employee aware of data quality and data ethics. Collaboration between BI and business teams must be strengthened to become a true partnership supported by agility.
A work in progress
Self-service analytics must support the business needs. The more the business teams will utilize data, the more needs will appear. Access to insights will lead to new questions and the identification of new opportunities. Therefore, the implementation of self-service analytics is a journey, where striking the right balance between innovation, pace of change, consistency, and knowledge management is key.
After a few years, some companies find themselves with a plethora of tools in their data architecture and a lack of clarity and control over their organization and shadow IT. Business users replicate similar requests, unaware of others’ similar approach. They have difficulties accessing the right data without a data dictionary in place, and little to no possibility to cross-reference data.
Some business users are increasingly accustomed to using intuitive, easy-to-use applications in their personal lives: they expect the same from self-service analytics solutions. They demand more control over data access and more control over data preparation. Combining their needs with those of a rationalization of the architecture and processing of data is therefore one of the issues faced by the IT teams.
In this journey, you need to be able to rely on people with enthusiasm and/or technical skills for self-service analytics, in a word: your champions. Done right, the approach of giving them the opportunity to regularly share challenges and best practices among each other and with other business teams will allow the company to create a learning dynamic, to support standardization and to build a knowledge base. This approach can take several forms, such as communities of analysts, user groups, and Centers of Excellence. Showcasing positive outcomes across the organization also helps build positive momentum and encourage adoption.
As previously exposed, the self-service analytics approach requires a true partnership between BI teams and business teams. As self-service analytics matures across the organization, this partnership will evolve and must be reflected in the data architecture and the governance, as well as in the organizational processes. BI teams provide support to users, monitor the data flows and the data infrastructure, and ensure the evolution of the overall architecture according to emerging needs and the required performance level.
Supporting your analytical journey in a nutshell
Taking self-service analytics to the next level implies going beyond deploying a tool and training your business teams on it. You should:
- Redefine the roles and responsibilities of your business teams.Make data literacy part of their new skill set.
- Make change management part of the process and rely on internal sponsors to help you carry out this project.
- Make sure to build solid foundations, such as a data governance program, and a reliable, documented, efficient and business-user oriented BI chain.
- Foster a real partnership between the BI team and the business teams. Create a positive group dynamic and rely on user communities, knowledge sharing and capitalizing on experiences.
Only then you’ll be on the fast-forward track to be data-driven and a self-service analytics champion.
Are you currently facing these challenges or are you interested to know more about how we support our clients in their self-service analytics journey? If so, feel free to contact us and we will be happy to elaborate together.
1Gartner defines data literacy as the ability to read, write and communicate data in context, including an understanding of data sources and constructs, analytical methods and techniques applied — and the ability to describe the use case, application and resulting value.
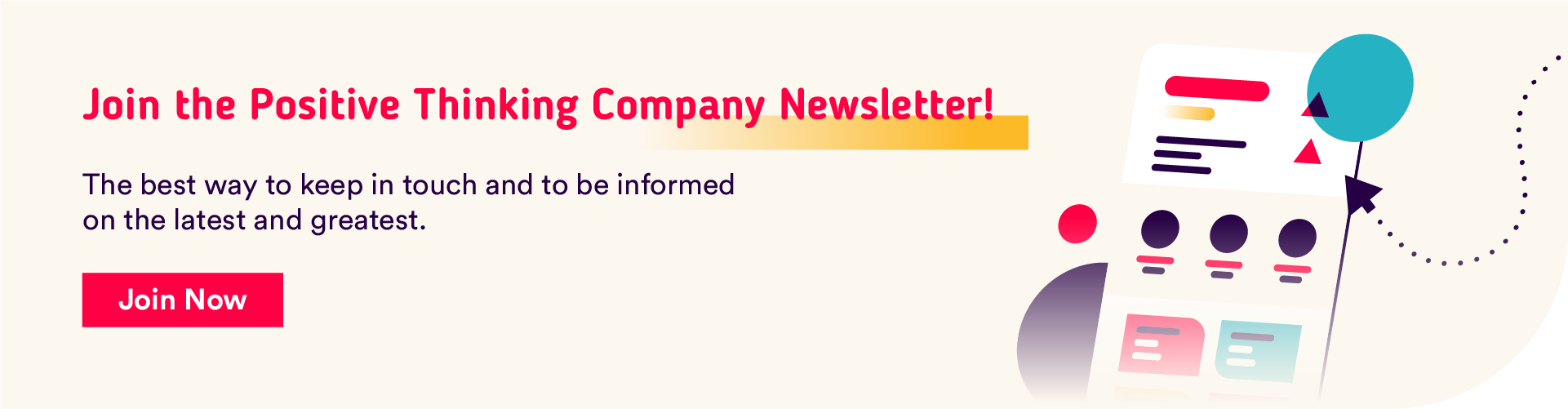