This article was updated on the EU AI Act agreement date, on December 9, 2023.
- Understanding the EU AI Act
- So, why do we need such a piece of legislation?
- A Risk-based Approach to AI Regulation
- Adoption of the EU AIA: A Significant Moment for the AI Environment
- The Importance of Explainable AI (XAI)
- What is XAI? What role does it play?
- XAI and Regulatory Compliance under the EU AIA
- Benefits of Leveraging XAI
- Practical Example: Meeting the EU AIA Requirements using XAI Techniques
- Challenges Companies Face in Implementing XAI for EU AI Act Compliance
- Understanding XAI’s Key Challenges
- The Trade-off Between Interpretability and Model Complexity
- The Need for Skilled ML Engineers, Data Scientists and AI Solution Architects
- Future Implications and Opportunities
- The Impact of EU AIA on Global Market Dynamics
- Key Takeaways
As we stand at the crossroads of the digital age, recent advances in Artificial Intelligence (AI) are shaping the future, promising incredible opportunities while also presenting complex challenges. One of them lies in the realm of regulatory compliance, with jurisdictions around the world enacting legislation to govern the use and application of AI technologies. With the EU AI Act officially agreed upon in December 2023, this article gains additional relevance in the evolving landscape of AI governance.
Among these, the European Union’s Artificial Intelligence Act (AIA) stands as a comprehensive regulatory framework that aims to ensure the ethical and responsible use of AI. In this article, we navigate the complexities of the EU AIA, shedding light on the role of Explainable AI (XAI) as a response to both simplifying and ensuring regulatory compliance. We dive into the essence of XAI, its benefits, and the challenges in its implementation, while also touching upon the future implications of the EU AIA on the global AI industry.
Understanding the EU AI Act
The European Union Artificial Intelligence Act (EU AIA) officially agreed upon in December 2023, represents a landmark legislative effort to create a comprehensive framework for governing AI systems within the EU. Initially introduced in 2021, this Act has now reached a pivotal stage in its journey towards implementation. Its primary goal remains to ensure that AI technologies are utilized ethically and responsibly, aligning with fundamental rights and societal values. The Act is a testament to the EU’s commitment to balancing the imperative of fostering AI innovation with the crucial need to address potential risks posed by these technologies to individuals and society.
So, why do we need such a piece of legislation?
The simple answer is that AI systems have become deeply integrated into our lives, influencing decisions in fields as diverse as healthcare, banking, employment, and more. While these systems offer incredible benefits, they can also have profound impacts, some of which may be harmful or discriminatory. The EU AIA is a response to these concerns, aiming to establish a legal framework that promotes transparency, accountability, and protection of individual rights in the face of AI’s growing influence.
A Risk-based Approach to AI Regulation
One of the key features of the EU AIA, as officially agreed upon in December 2023, is its nuanced, risk-based approach to AI regulation. The Act categorizes AI systems into the following levels of risk:
- Minimal Risk: This category includes AI systems that pose minimal risk to safety and fundamental rights. These systems are generally subject to minimal or no specific legal obligations, allowing for innovation while ensuring basic safety standards.
- High Risk: AI applications in this category have significant potential to cause harm or adverse effects due to their involvement in critical sectors. This includes AI used in critical infrastructure, education and vocational training, employment, essential private and public services, law enforcement, migration, asylum and border control, and administration of justice. High-risk systems are subject to stringent regulatory obligations before they can be deployed, ensuring a high level of safety and compliance with fundamental rights.
- Unacceptable Risk: AI systems that pose a clear threat to safety, livelihoods, and fundamental rights are categorized as unacceptable risk and are banned outright. This category might include AI systems that manipulate human behavior, exploit vulnerabilities of specific groups, or otherwise lead to physical or psychological harm.
- Specific Transparency Risk: This category addresses AI systems that require specific transparency obligations. It includes systems where it is crucial for users to know that they are interacting with AI, ensuring clarity and preventing deception or confusion.
These risk categories within the EU AIA ensure that the potential harms posed by AI systems are proportionally addressed, creating a safer and more trustworthy environment for their deployment and use. The inclusion of specific transparency risk as a category highlights the EU’s commitment to user awareness and ethical AI practices.
Adoption of the EU AIA: A Significant Moment for the AI Environment
In a significant development, the EU AI Act was officially agreed upon in December 2023, marking a major milestone in the EU’s approach to AI regulation. This follows its initial adoption by the Internal Market Committee and the Civil Liberties Committee, which demonstrated a strong majority in favor and underscored the urgency and relevance of this comprehensive legislation.
The Act expands the classification of high-risk areas to include harm to people’s health, safety, fundamental rights, or the environment. AI systems that influence voters in political campaigns and recommender systems used by social media platforms with more than 45 million users under the Digital Services Act have been added to the high-risk list.
The Act includes obligations for providers of foundation models, like GPT, who would have to guarantee robust protection of fundamental rights, health and safety, the environment, democracy, and rule of law. They would need to assess and mitigate risks, comply with design, information and environmental requirements, and register in the EU database. They would also have to comply with additional transparency requirements, like disclosing that the content was generated by AI, designing the model to prevent it from generating illegal content, and publishing summaries of copyrighted data used for training.
To boost AI innovation, the Act has added exemptions for research activities and AI components provided under open-source licenses. It also promotes regulatory sandboxes, or controlled environments, established by public authorities to test AI before its deployment. The Act also strengthens citizens’ right to file complaints about AI systems and receive explanations of decisions based on high-risk AI systems that significantly impact their rights.
Earlier in 2023, the co-rapporteurs of the Act, Brando Benifei and Dragos Tudorache, have expressed confidence that this Act balances the protection of fundamental rights with the need to provide legal certainty to businesses and stimulate innovation in Europe. They emphasized the importance of building citizens’ trust in the development of AI and the opportunity for the EU to lead the way in making AI human-centric, trustworthy, and safe.
With the official agreement in place as of December 2023, the EU AIA is now set to move into the implementation phase. This transition marks a shift from legislative development to practical application and enforcement, setting a new standard for AI regulation in Europe and beyond.
Recognizing the importance of this act, we fully endorse its role in AI regulation. Evolving in the data and AI landscape for many years, our focus is on proactively responding to these changes and supporting organizations to do the same. A key part of our strategy is a significant investment in XAI know-how. Let’s now uncover the ways in which XAI can tackle regulatory compliance and serve as a compass for organizations to navigate through the labyrinth of the AIA.
The Importance of Explainable AI (XAI)
In a world increasingly driven by AI, the ability to understand and interpret the decisions made by AI systems has become crucial. This has led to the emergence of XAI, a field that strives to make AI decision-making transparent and understandable. As we navigate the complexities of the EU AIA, the role of XAI becomes even more significant.
What is XAI? What role does it play?
XAI refers to methods and techniques in the application of AI where the results of the solution can be justified to human experts. It is an offshoot of AI focused on creating a clear, understandable pathway between data input and output, thereby promoting transparency.
The transparency offered by XAI is critical in maintaining accountability in AI systems. When an AI system makes a prediction, particularly one with significant consequences, it’s important for users, regulators, and affected parties to understand how that decision was made. By providing clear, accessible explanations of AI processes, XAI allows for meaningful human oversight and accountability.
XAI and Regulatory Compliance under the EU AIA
XAI is not just a good-to-have feature; it’s a crucial requirement for regulatory compliance under the EU AIA, especially but not solely for high-risk AI systems. The EU AIA mandates that high-risk AI systems must provide clear and comprehensible information about their capabilities and limitations, and that their decision-making process should be transparent and traceable. Without applying XAI, meeting these requirements would not just be challenging, it would be almost unfeasible.
Companies deploying AI solutions, such as customer service chatbots and product recommendation systems, fall under the “limited risk” category as per the EU AIA. Such companies are obligated to ensure transparency, clearly indicating when users are interacting with an AI system and informing them about the capabilities and limitations of these systems.
While stringent regulations do not apply to “limited risk” AI systems, there is a compelling business case for these companies to adopt XAI. By doing so, organizations can gain insights into how their AI systems arrive at decisions and recommendations provided to their customers. This understanding is crucial to both the improvement of AI systems and the confidence of the customers interacting with them. With XAI, these companies can refine their AI systems, ensuring that they align with business objectives, customer expectations, and regulatory guidelines. Thus, even in the context of “limited risk” AI systems, XAI offers distinct advantages that can enhance business performance and customer satisfaction.
Benefits of Leveraging XAI
While the importance of XAI in regulatory compliance is clear, the benefits of adopting XAI extend far beyond compliance. Embracing XAI can lead to significant advantages, both for organizations deploying AI systems and for the individuals who interact with them:
- Building Trust: Transparency in decision-making is a powerful tool for building trust. When AI systems can explain their decisions in human-understandable terms, it reassures stakeholders that decisions are not made arbitrarily, but based on a clear and fair analysis. This can help improve the acceptance and adoption of AI systems.
- Mitigating Risks: XAI can help organizations uncover and understand potential issues with their AI systems, such as biases or inaccuracies. By understanding the “why” behind AI decisions, these problems can be identified and addressed proactively, mitigating potential risks.
- Supporting Continuous Improvement: By making the inner workings of AI systems understandable, XAI opens the door for continuous improvement. Insights gained from XAI can guide developers in refining models, improving accuracy, and enhancing overall system performance.
- Facilitating Better Decision-Making: In many contexts, AI systems are used to support human decision-making. By providing clear explanations for their output, XAI systems can give humans the context and understanding they need to make better-informed decisions.
- Bolstering Ethical AI Practices: XAI aligns closely with the principles of ethical AI, including fairness, accountability, and transparency. By adopting XAI, organizations can demonstrate their commitment to ethical AI practices, enhancing their reputation and standing in the market.
In essence, adopting XAI is not just about meeting regulatory requirements—it’s about harnessing the power of AI in a responsible and effective manner, leading to better outcomes for all.
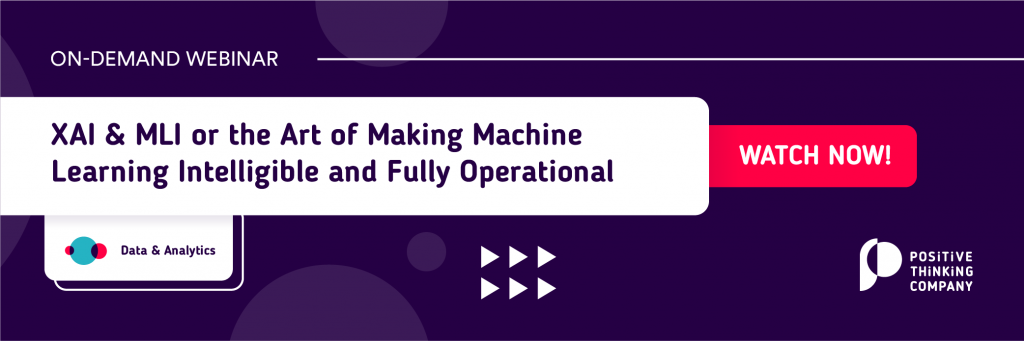
Practical Example: Meeting the EU AIA Requirements using XAI Techniques
Consider a high-risk AI system used in hiring processes. This system analyses a vast amount of data – including applicants’ qualifications, experience, skills, and interview responses – to recommend the most suitable candidates for a job.
By leveraging XAI techniques, the system can provide clear explanations for its recommendations. For instance, it could highlight that it suggested a candidate because their skills and experience closely match the job requirements. In case the system rejects a candidate, it could explain the factors contributing to this decision, such as a lack of necessary skills or experience.
Or in the undesirable case, and as we have seen in the past, highly skilled people were rejected although their skills matched the job requirements perfectly. The AI recruiting system made its decision based on gender because similar positions were filled by men in the past and the AI system “thought” that gender was important for the decision. Without XAI techniques, the company would not have been able to find out about this (serious) issue and would have allowed discrimination based on gender.
In this way, XAI allows the organization to demonstrate transparency and accountability, as required by the EU AIA, while also providing valuable insights that can be used to improve the AI system and the hiring process.
Challenges Companies Face in Implementing XAI for EU AIA Compliance
It is a fact that XAI comes with plenty of challenges. From technical difficulties to human resource requirements, organizations will need to overcome several obstacles to successfully implement XAI.
Understanding XAI’s Key Challenges
While the benefits of XAI are undeniable, organizations might face several challenges when implementing it:
- Model Complexity vs. Interpretability: XAI seeks to make AI decision-making transparent and understandable, but this goal can be at odds with the complexity of advanced AI models. Deep learning models, for instance, are known for their accuracy but are notoriously hard to interpret.
- Maintaining Performance: Achieving explainability often comes with a cost to performance. Simpler, more interpretable models may not perform as well as their complex counterparts. Balancing the need for explanation with the desire for high-performing models can be a delicate act.
- Lack of Standardization: XAI is a relatively new field, and there’s a lack of standard definitions and methods. This can make it difficult for organizations to know which approaches to adopt and how to measure their success.
- Data Privacy: XAI techniques often require access to more detailed data to provide explanations. This can raise privacy concerns, particularly in sectors dealing with sensitive information.
- Resource Requirements: Developing and deploying XAI solutions require a team of well-trained Machine Learning (ML) Engineers and Data Scientists. Finding and retaining such talent can be a challenge.
The Trade-off Between Interpretability and Model Complexity
One of the most significant challenges in XAI is the trade-off between model complexity and interpretability. Complex models, like deep neural networks, can capture intricate patterns in data and often provide superior performance. However, their inner workings can be difficult to interpret, likened to a ‘black box’. On the other hand, simpler models such as decision trees or linear regression are more interpretable but might not perform as well on complex tasks.
The challenge for organizations is to find a balance: to develop AI models that are both powerful enough to deliver accurate results and simple enough to be understandable. This might involve using hybrid approaches, developing new XAI techniques, or accepting certain trade-offs in either performance or explainability.
The Need for Skilled ML Engineers, Data Scientists and AI Solution Architects
Implementing XAI isn’t just a technical challenge—it’s also a human one. Designing, developing, deploying, and maintaining XAI projects require a team of skilled AI Solution Architects, ML Engineers and Data Scientists. These professionals need to understand not just AI and ML, but also the principles of XAI and the regulatory requirements of the EU AIA.
However, the demand for such professionals often outpaces supply, and competition for talent can be fierce. Organizations will need to invest in training and development, or partner with external experts, to build the skills needed for successful XAI implementation.
Even with the challenges it presents, the advantages of XAI in regulatory compliance, risk mitigation, and trust-building position it as a valuable investment for organizations using AI systems.
Future Implications and Opportunities
As we look towards the future, it is clear that the adoption and implementation of XAI will have far-reaching implications and opportunities, not only within the European Union but globally. The AIA has not only influenced the AI industry within its jurisdiction but has also sent ripples across the globe, influencing market dynamics and the regulatory landscape of AI.
The Impact of EU AIA on Global Market Dynamics
The AI landscape has been significantly shaped by the EU AIA, leading to a reshaping of the global market dynamics. This can be most clearly seen in the stark contrast between the different interpretations of AI regulations in the United States (US), China, and the EU.
For years, the US had a laissez-faire approach to AI regulation, reflecting a general reluctance from both legislators and tech giants. However, the rising impacts of unregulated social media and the tremendous power of AI Large Language Models (LLMs) have highlighted the urgent need for oversight. This has sparked an evolution in the attitudes of industry figures and legislators alike, including prominent voices like Sam Altman (CEO of OpenAI) although we can not know his true intentions, advocating for AI regulation and the formation of international standards.
Despite various attempts to introduce regulation, including proposed bills like the Algorithmic Accountability Act and the American Data Privacy Protection Act, federal legislation on AI remains absent. The growing recognition of the need for technical expertise in regulatory agencies, and strong enforcement powers, has stirred discussions around a new digital regulator for overseeing social media companies. Today, bipartisan support for comprehensive AI regulation is seen as crucial, with the overarching goal being responsible AI development and use.
Conversely, China has a strong governmental role in AI regulation. The Cyberspace Administration of China (CAC) released stringent regulations in April 2023 outlining rules that AI companies need to adhere to, including the requirement for security reviews before an AI model is publicly released. The regulations, which align AI development with China’s socialist values, hold AI companies responsible for the information generated by their software.
The differences in AI regulation between these global players create a complex and dynamic global market environment, fueling competition and innovation, but also raising concerns about privacy, ethics, and societal harm.
As the regulatory landscape continues to evolve, it will be critical for businesses to not only comply with these regulations but also understand how they can leverage XAI to gain a competitive advantage or mitigate potential disadvantages.
Key Takeaways
- EU Regulation: The EU AIA, officially agreed upon in December 2023, is a comprehensive regulatory framework for AI systems in the European Union. It categorizes AI systems into three risk levels: unacceptable, high risk, and low to minimal risk. Each level has different requirements, with high-risk AI systems now encompassing a broader scope, including potential impacts on health, safety, fundamental rights, or the environment. These systems are subject to strict rules related to transparency, human oversight, and robustness.
- Risks of Non-Compliance with EU AIA: Non-compliance with the EU AIA could result in significant penalties, including fines up to 6% of global annual turnover, reputational damage, loss of customer trust, and operational disruptions due to enforcement actions.
- Importance of XAI: XAI is crucial for transparency and accountability in AI systems. It aids in understanding the decision-making process of AI models, which is vital for regulatory compliance, building trust, and improving model performance. XAI also serves as a tool to comply with the EU AIA regulations, as demonstrated by the hypothetical case of a bank using XAI to provide loan decision explanations.
- Challenges in Implementing XAI: Companies face several challenges in implementing XAI, including the trade-off between model complexity and interpretability, the need for well-trained AI solution architects, ML engineers, and data scientists, and technical difficulties in achieving explainability without sacrificing performance.
- Global Impact of EU AIA: With its official agreement in December 2023, the EU AIA is poised to significantly influence the global AI industry and regulatory landscape. The Act’s far-reaching implications and the global nature of many tech companies may lead to shifts in AI regulation approaches worldwide, potentially affecting geopolitical dynamics.
- Opportunities and Challenges with XAI: Embracing XAI can provide competitive advantages such as increased trust, regulatory compliance, improved decision-making, and customer engagement. However, it also comes with challenges like managing model complexity, ensuring data privacy, bearing the costs and time for implementation, dealing with a limited pool of XAI experts, and navigating regulatory uncertainty.
NB: This content was produced with the support of generative AI under the guidance of our authors.
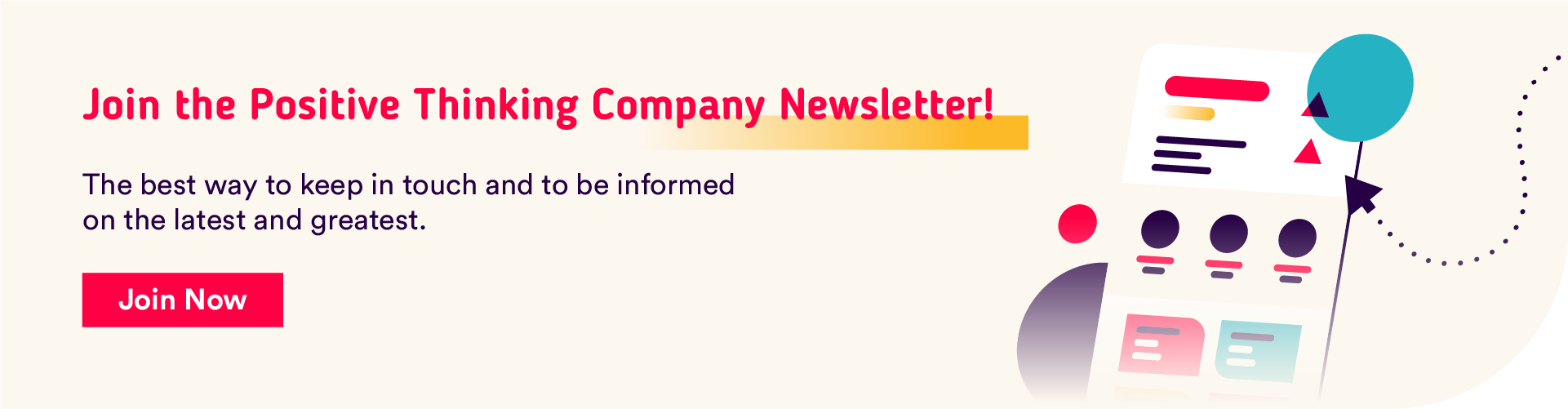