This case study details how we built an automated data quality management system solution to address trust issues with the data and maximize both the return on investment of Publicis Media in a centralized data platform and the quality of its BI and analytics activities. Discover how we achieved a 50-70% reduction of issue detection and mitigation time while regularly profiling 100% of the data implemented.
- Data Governance
- Data Quality
- Data Products
- Data Quality Management
- Data Quality Automation
- Business Intelligence & Analytics
- Media
Key Challenges
Our client is one of the largest multinational advertising service providers and media groups. It offers various communication services. Within the scope of performance analyses, the company collects large amounts of data from various advertising platforms. All of this precious information is stored and transformed in a unique data platform which aims at providing actionable data to each business team.
However, our team of Data Engineers working on this data platform noticed some technical and functional quality problems. These could only be detected and corrected manually. And when there are data quality issues, there are inevitably trust issues with the results of the analytics, reporting and data science activities being used by the business teams.
In this context, our client wanted an automated quality assurance solution that would:
- provide transparency about the data quality during the data integration process,
- automatically eliminate simple data problems and identify more complicated cases,
- provide monitoring for technical and business users.
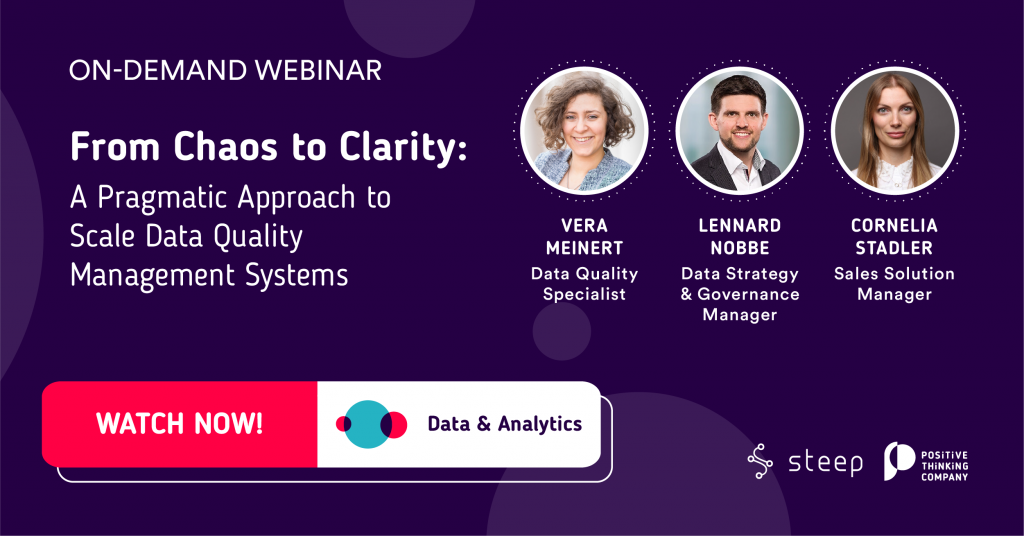
Our Approach
In order to address our client’s challenges, we:
- Analyzed the current situation including the data quality checks already performedand we collected all the stakeholders’ requirements (both from technical and business teams).
- Evaluated the potential for automation and generalization of existing quality assurance initiatives.
- Designed a quality assurance module that fits into the existing platform infrastructure and complements it with important components. We worked on three quality gates: a first check on the raw data, a second check after the harmonization phase and a final check on the data to be delivered/provisiones to the teams/data products.
- Implemented these technical and functional quality checks for initial data sources and projects to provide an MVP
- Created generalized quality levels (depending on the data sources and the expected quality delivered by the source) that can be communicated and understood easily.
- Implemented new roles (e.g. Quality Assurance Product Owner, Owners for the specific quality checks, Data Stewarts) and processes (e.g. RACI matrix)complementing the quality assurance solution.
- Implemented a reporting system in the form of dashboards and notifications for monitoring the data quality based on the checks.
- Scaled the automated quality assurance solution to further data sources and other projects.
- Coached and trained the business and technical teamsin use and operation. It enabled us to collect new inputs in order to further improve the whole quality assurance system in place.
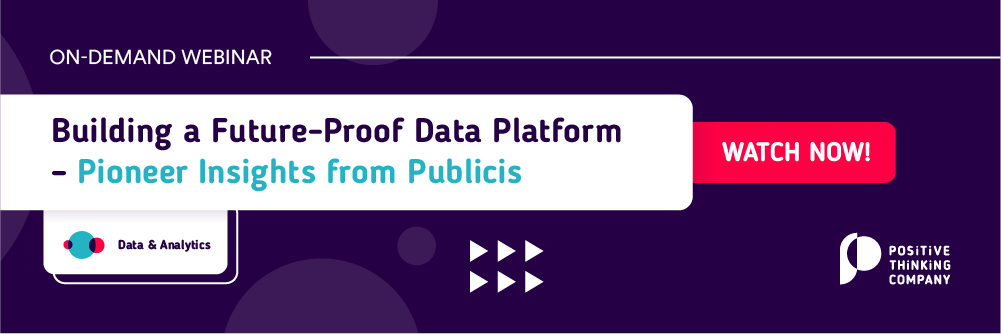
Benefits
- 50-70% reduction of issue detection and mitigation time.
- The data being used and provided by our client is of high quality.
- There is full transparency about the quality of the data being used by the business and technical teams.
- Only three years till full amortisation of the automated data quality management system implemented.
- 100% of data of implemented data sources is profiled regularly and any quality issue with new data sources or approvals can be quickly identified, thus improving the quality of the BI and analytics activities of Publicis Media.
- The trust in the data platform strongly increased and new users are leveraging its quality data every day. Publicis is thus increasing its return on investment in this unique platform, and the teams are not using third party tools and performing manual tasks anymore.
Teams involved on this project
A Product Owner and a Data Engineer collaborated with our client on this project for almost one year.
Technologies and Partners

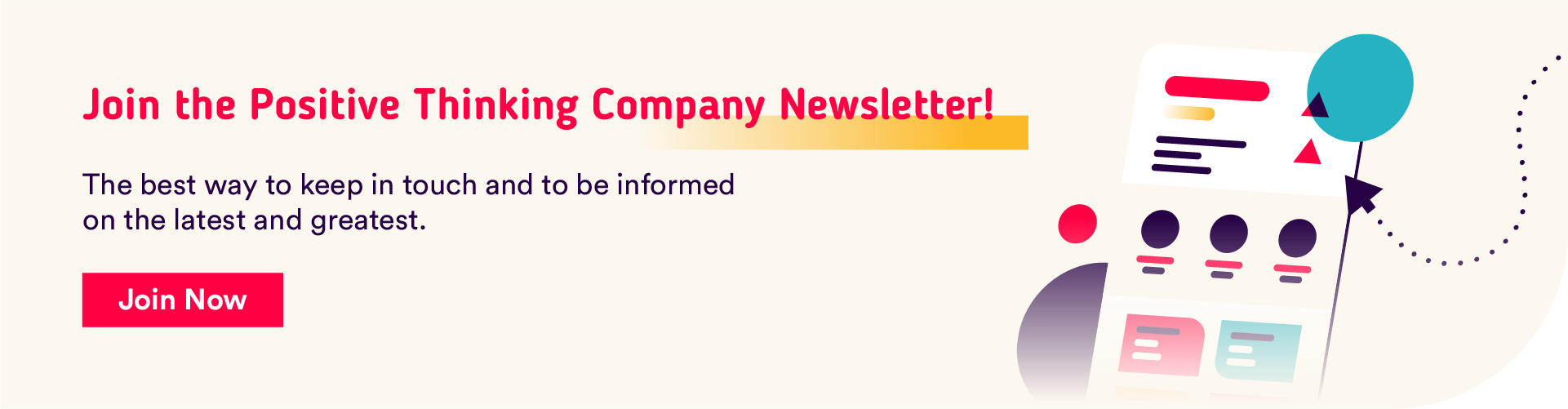