The first thing you learn, when you start to study Data & Analytics? “Garbage in, garbage out”!
Believe it or not: every data student would tell you this at first when you call him or her in the middle of the night and ask about the most known pitfall of data products.
This common phrase is used to clarify one of the most known paradigms: If you don’t have the necessary data in high quality, you will not get high-quality models and analytical solutions with high accuracy and relevancy for your business problem(s).
High data quality is one of the key success factors for high-value analytics projects. It is the base for all further developments of a use case. If you don’t have good data, you don’t even need to start your project. By ensuring data with high quality, you have a good chance that your project will have a high-value outcome.
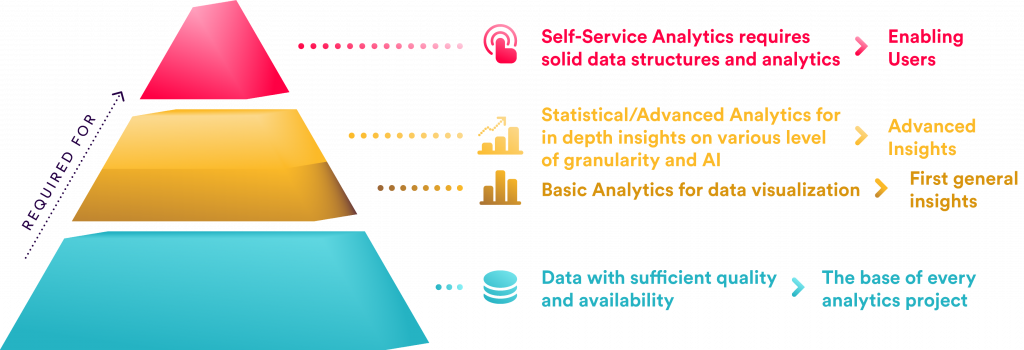
Why is data quality more important than ever?
But when every master student already knows, why is it more important than ever to talk about this topic?
- The total amount of data being captured and stored by industry doubles every 1.2 years
- Every two days we create as much information as we did from the beginning of time until 2003
- The annual costs of data quality issues in the U.S. were estimated to amount to $3.1 trillion in 2016
- The costs of dealing with the business problems caused by bad data are estimated to be in the range of 15% to 25% of companies’ annual revenue on average.
That’s why! The topic is never getting old – nowadays the problem to assess the quality of these huge amounts of data is even more relevant than before. Ignoring this might lead to significant costs and downstream business problems.
What does data quality actually mean?
Depending on the context and usage of the data you might want to define different quality criteria and requirements. Common criteria are:
- Timeliness: Is your data up to date and the necessary time range available? (e.g. do you have the information available till today?)
- Completeness: Is your data complete or does it contain missing values or even missing records? (e.g. is the address of a customer missing?)
- Accuracy: Is your data correct and accurate in reflecting the reality? (e.g. does the postal code of a customer exist and match the city?)
- Consistency: Is your data consistent over different data sources? (e.g. is the address in your CRM system matching the address in your sales tracking system?)
- Validity: Is the data available in the expected format? Are business rules fulfilled? (e.g. is a phone number saved with the country code?)
- Uniqueness: Are there duplicates in the data base? (e.g. double entries of customers caused by name changes due to marriage)
There are a lot more criteria you might want to check in your daily business, but this is very subjective for your company and probably even the business use case you want to cover. It’s always hard to define (generic) quality metrics under these circumstances. But in the end, you really need to take your specific demands into account. Monitoring and improving the data quality in your organization starts with a specific definition of quality and its metrics. Combining this with the right tools and technologies to assess the data quality, track quality issues and finally set up processes for the mitigation of those issues will lead to higher data quality!
What are the biggest challenges that will cross your path to higher data quality?
Sounds easy? Yeah right – but unfortunately there are a couple of challenges you will phase when it comes to data quality. From our perspective the three main ones are those below:
Challenge n°1: Definition of quality metrics
One of the biggest challenges is for sure the definition of quality metrics. What does it mean if you have a data set with high-quality data? Do you want to choose a generic rating that will exactly tell you which requirements are met and to what extent? Or rather a flexible one that assesses the quality with respect to the abilities of the source? Or even the specific requirements per use case? All sides have their pros and cons! It totally depends on the data sources you are using, the level of automation in your data collection process and finally on the bigger topic of Data Governance and its implementation in your organization.
Challenge n°2: Definition of quality rules
Second, the definition of quality rules can be challenging: Defining data quality requirements as a strict set of rules makes the assessment of data quality quite easy but holds some pitfalls. In case of new requirements or significant changes in the quality of the raw data, you will have a lot of effort to adapt your rules accordingly. It’s for sure the way to start with but over time it makes sense to address data quality with more flexible ratings (based on statistical indicators) or to even install machine learning models to assess the data quality (e.g. to predict the expected value of a missing value or for verification reasons).
Challenge n°3: Definition of a quality process
Finally, the needed level of automation of your data quality assurance process can be hard to define. It depends on how specific your requirements are, how much data you process for your use case and if data is reused. Are we speaking about a data quality solution for a fully automatized data platform with giga bytes of data being processed every day from multiple API-based data sources used for different reporting and data science use cases? Or do you want to check the manually collected data of a process where you want to reduce the rejects of a manufacturing pipeline? Full automation needs more development time but might be totally worth it when you save a lot of time for multiple people in their daily working environment.
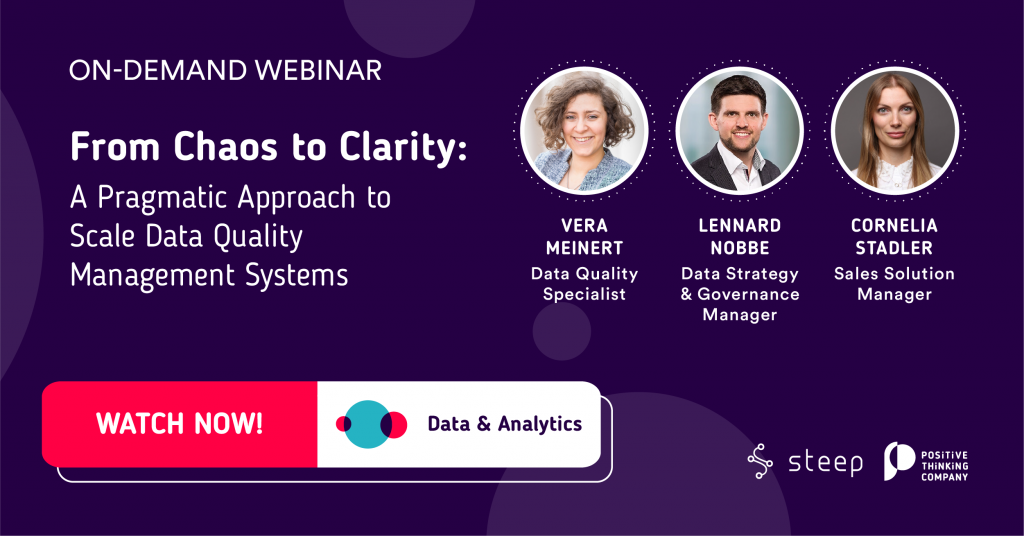
How can you ensure high data quality in your organization?
How can you ensure now that the expected standards are met in your organization?
First, start with defining solid quality metrics that fit your needs. Combining them with quality requirements you can assess the current level of quality of your data.
Next, define roles and processes around the quality initiatives. You need people taking ownership of the data quality solution itself as well for the data sources and their requirements. Installing the matching processes around the solution will keep things organized and compliant.
Now, you are ready to set up a monitoring system to assess your data quality over time and track issues as fast as possible. So finally, you are ready to mitigate quality issues and to improve your data quality on the long run.
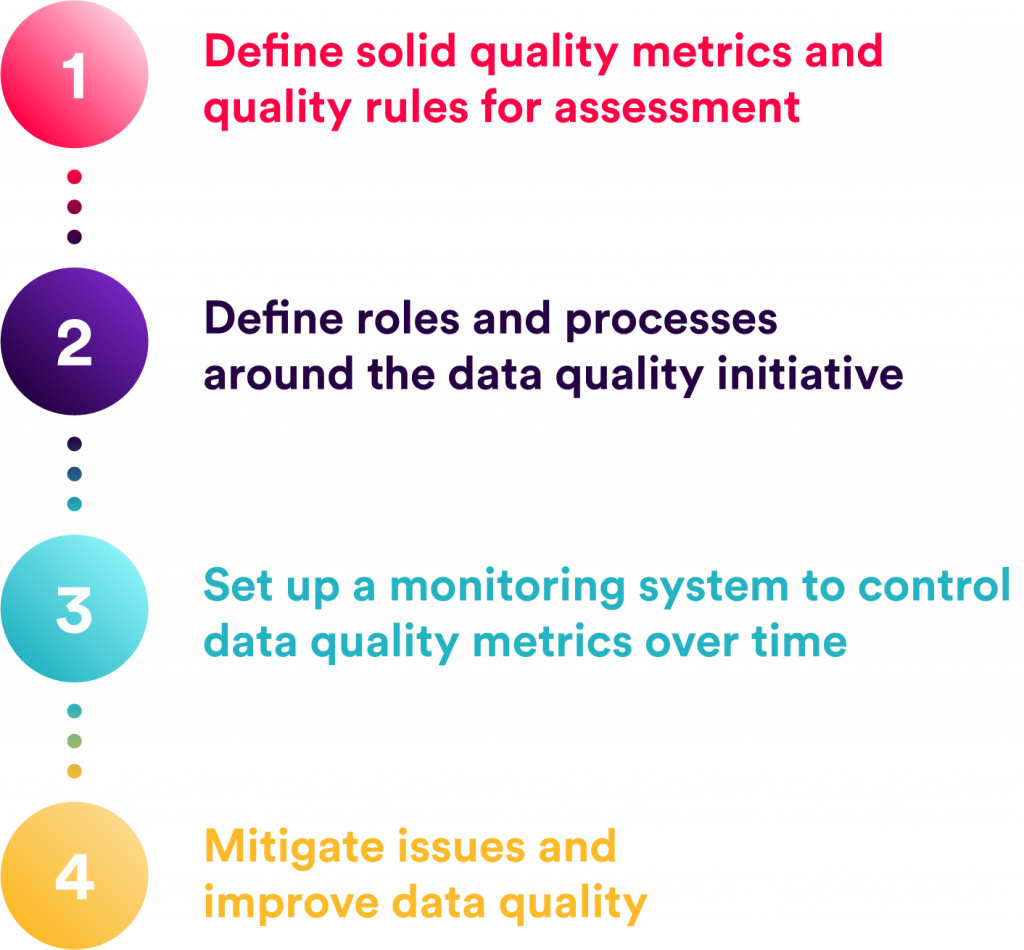
And, to make your initiative really successful make sure that you:
- Create a flexible and transparent system that is open for iterative improvements of all key aspects. Let it be quality metrics, automation level or the level of intelligence of your rule.
- Include experts on data governance and on technical implementation as well as data engineers and data scientists.
- And finally: Get started! It’s time to turn the common saying around: “High value in, high value out!”
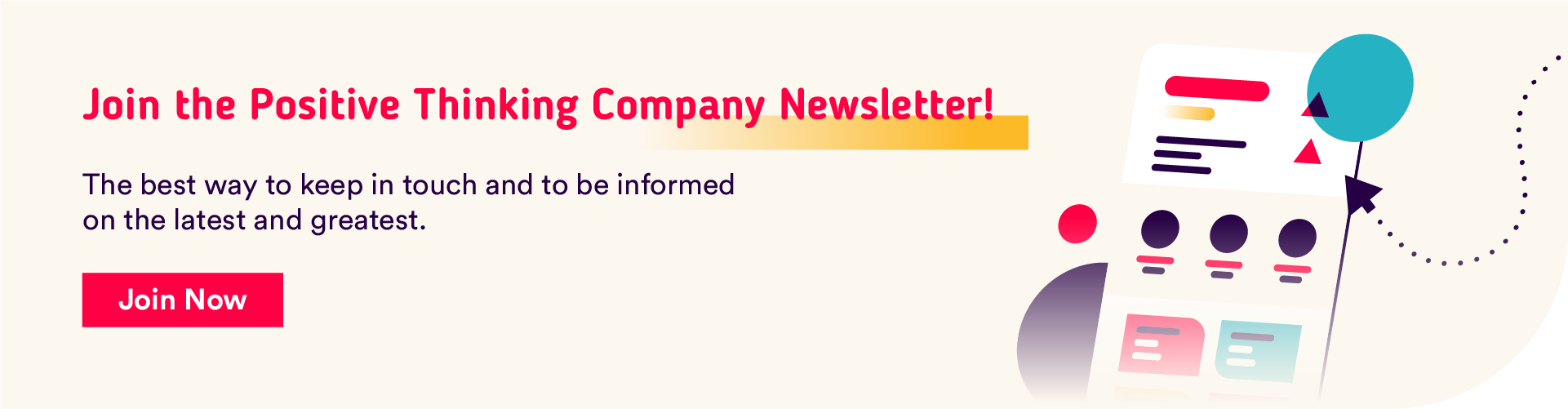