- Wealth Management
- Data Science
- Organizational embedding
- Customer Analytics
- Account Management
Our customer
Our customer, Julius Bär, initiated its own data science start-up and innovation laboratory under the name “Target Insights” in spring 2018, with the aim of using available data more efficiently – for example to improve customer service – and promoting a data-driven culture. In addition to finding and implementing profitable analysis scenarios, another key objective is to build up internal knowledge and experience.
Key challenges
Client relationships in asset management are particularly complex, which is why a deep understanding of these relationships and a high level of client knowledge are important differentiating factors in competition. Since data science projects typically require cross-departmental cooperation, the usual organisational structures with their usually strict separation between IT and specialist departments are conceivably unsuitable. Such projects therefore typically fail due to conflicts of competence, individual interests and a lack of willingness to cooperate rather than due to a lack of technical competence. An important step towards the success of data science projects is therefore the decision for a suitable, easily expandable team structure with clear roles and responsibilities.
Our approach
As this is a customer analytics application, it is essential that people with customer contact as well as experts from the relevant departments are already involved from the beginning of the project in order to identify as many ideas for analysis scenarios as possible. Here it proved to be an advantage to integrate selected domain experts from Julius Bär directly in the data science team, who discuss, collect and validate analysis scenarios together with the customer service representatives.
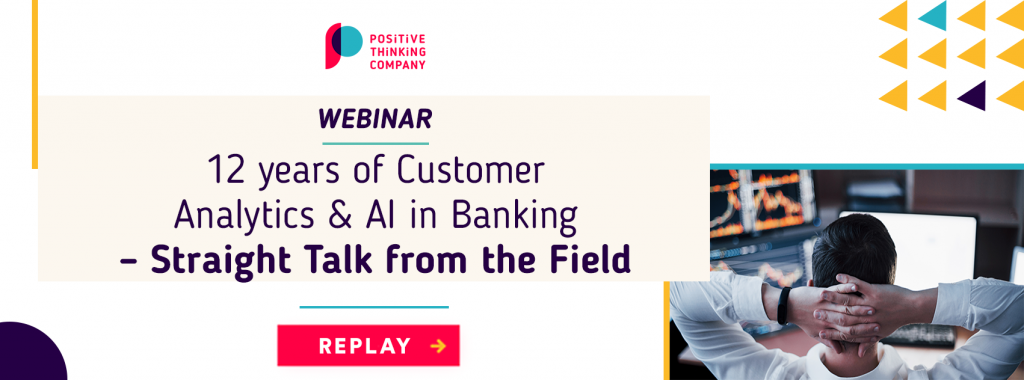
After selecting an analysis scenario, the subtasks of data acquisition, exploration and pre-processing have to be performed. The designated roles of data architects, data analysts and data engineers work closely together with the data scientists, who are exclusively responsible for the actual data analysis.
In this given project, we decided to choose the following analysis scenario: Is there a reliable sequence of early warning indicators over the course of the customer lifecycle that can be used to predict when the strength of a customer relationship – and thus customer satisfaction and loyalty – will decrease to such an extent that action by the customer advisor is recommended?
Benefits
For account managers at the Zurich headquarters of Julius Bär, the action recommendations described above for increasing customer satisfaction are already being played out regularly, after they have been trained in the use of the system in several extensive coaching sessions. In recurring feedback loops, user feedback on the current action recommendations is now collected in order to further improve the quality of the models. The experience gained in this process has already been used to create separate forecast models for other locations, which have now also been transferred to productive use.