This case study details how we helped one of the largest energy suppliers in Germany and Europe to set-up a rigorous automation of their data science process in order to maximize the return on investment.
- Natural Language Processing (NLP)
- Natural Language Understanding (NLU)
- MLOps
- Cloud
- Process Automation
- Low Code
- Microservice Architecture
- Data Science and AI
Key challenges
Our client is one of the largest energy suppliers in Germany and Europe. They provide more than 5.5 million people with gas, power, water and energy-related services and products.
Operating in a highly homogenous market by nature, our client identified customer service quality as a key differentiator. They started to and continue to utilize Natural Language Processing in order to increase customer support efficiency and quality.
Our client wanted to build a platform that:
- Facilitates efficient operationalization and reliable as well as robust operation of Natural Language Processing – currently the main Use Cases;
- Is highly flexible and adaptable to new business challenges in the shortest time possible;
- Enables business-focused people to integrate their process knowledge into the platform.
Our approach
We help our client to achieve their goal in three ways:
- Setting up a highly automated MLOps environment.
We started by building up a Data Science Lab to cover the whole Data Science Process. By using dedicated MLOps tools, we ensured model comparability as well as reproducibility of data, training and resulting models. At the same time, we maximized the development efficiency by rigorously automating model training, evaluation and deployment. - Building various NLP microservices.
After having built the Lab, we utilized it to build and continuously improve various Natural Language Processing microservices capable of domain-specific text classification and information extraction tasks. - Enabling and training business-focused teams to automate business processes.
While we own the technical expertise, our client masters process knowledge. To utilize this, we set up a low-code environment. It enables business-focused employees to combine and orchestrate the provided NLP services in order to automate business processes while simultaneously integrating their expertise into these automations.
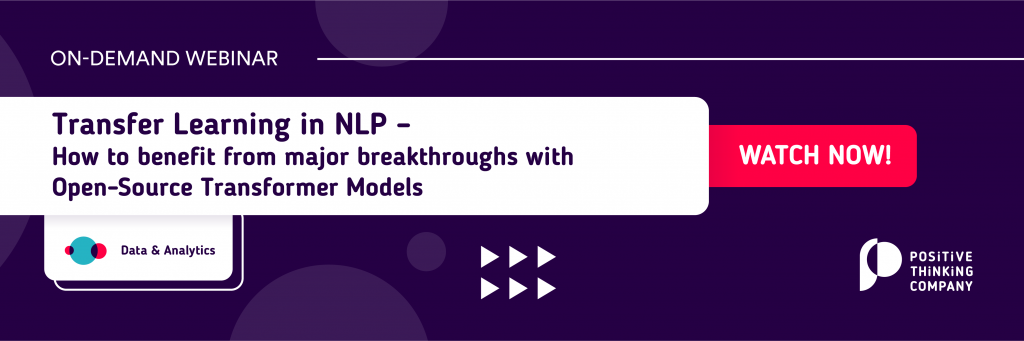
Benefits
- Ready-to-use data science platform perfectly adapted to our client needs;
- Operationalization of more than 50 models within 22 months;
- Automation of seven processes so far and still counting;
- Maximization of the efficiency of training and deploying NLP models;
- Optimization of the collaboration between Data Scientists, Cloud Engineers and Business Functions;
- Enablement of our client’s teams to independently automate processes while using the created capabilities.
Team involved
Data Scientists, ML Engineers, Cloud Architects, DevOps Engineers and a Project Manager collaborated with our client for 22 months.
Technologies & Partners

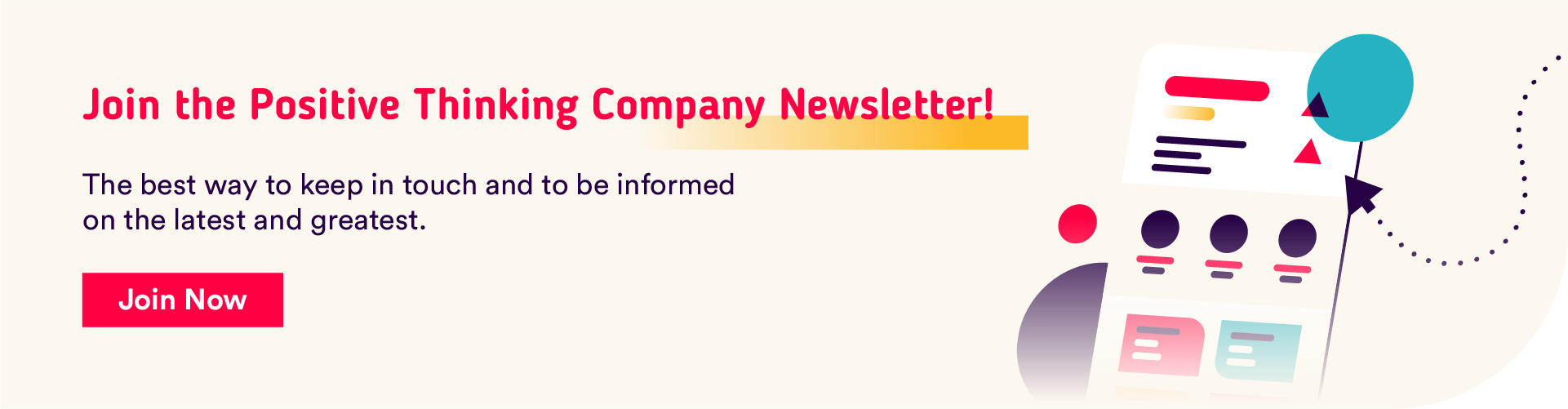