Daoud Chami, Innovation Solution Manager at Positive Thinking Company on Data & Analytics expertise, questions the evolution of the Data Scientist profession.
This article is based on a talk by Daoud, ‘Where is Data Science going?’, given during a Meetup with Lyon Data Science and Devoxx 2023.
This is a meta subject, accessible for a broad audience, because it does not require to be technically familiar with Data Science to understand it.
The goal is to answer this question, which may seem easy at first: What is the future of the Data Scientist profession?
Indeed, in the current context, customers want to design products and not just POCs, with more platforms and infrastructures that can manage a whole part of devOps, from production to model lifecycle management. On the other hand, we also hear a lot about a new term: Machine learning engineering.
In view of these changes, how will Data Scientists have to position themselves on the Data value chain?
To answer this, Daoud reviews the basics of the Data Scientist profession, the scope of the expertise on various Data professions and finally the future possibilities of this profession.
What differentiates Data Scientists from other Data professions?
The Data Scientist is the one who trains a model, starting from data and mobilizing business skills.
A model is an algorithm whose behaviour depends on parameters that can be estimated during the training phase on a large data set to produce predictions that are as faithful as possible to the expected end results. Basically, it is about predicting something.
The Data Scientist is either the one who designs the model or the one who writes the code that produces the model.
Of course, the Data Scientist doesn’t just do that. But this is what distinguishes him from other jobs related to Data. This is where his core ability and added value lies.
For example, with a dataset composing multiple variables to describe real estate, a data scientist’s mission may be to predict the price based on certain variables. To answer the question: Are we able to predict the price of a property from its description data?
The basic assumption is that there are correlations between these variables and the asking price. The Data Scientist will then check the links between each variable and the price of the apartment. Furthermore, he will also perform feature engineering, to combine information and create a new result. Lastly, he will check if this latest information also makes it necessary to act upon.
This will of course require business knowledge on the sector concerned.
The job of the Data Scientist is therefore to collect information and create information when it is not available yet, which will be necessary to learn, and make predictions.
By mobilizing business and mathematical knowledge, he can propose a model, which will have to be put into production further down the line.
To do this, the Data Scientist will mobilize mobilize probabilities to help his prediction.
Here, we can already see a major difference compared to a regular Data Analyst, who will instead mobilize statistics to meet end results.
Indeed, the Data Scientist will run essentially on the following chain: Data cleaning, extraction or creation of relevant features, training of a model, and its evaluation. This cycle is iterative and can be repeated as many times as necessary until a model is obtained, when possible, that predicts well enough what one is trying to describe.
However, a Data Scientist does more than that. There are also some who are adept at doing data analysis and even using Tableau, Power BI,… There are also Data Scientists who are good at data engineering: Preparing data, formatting it and storing it in the most optimized way possible on distributed systems.
Machine learning is therefore the ability that differentiates Data Scientists from regular Data Analysts, but it doesn’t entirely characterize them. The Data Scientist does machine learning, and sometimes Data Analysis and Data Engineering. Sometimes, he will also deploy and manage the CI / CD, to adapt the life cycle of machine learning products to more complex systems as well as for more basic systems. This also implies rigorous testing and configuration, but our Data Scientist should be polyvalent. It is rare however that one masters all these factors and does everything himself.
What are the scope the Data Scientist and other Data professions?
Overall, we have gone from a vision of the Data Scientist’s profession as represented by the Venn diagram on the left (an expertise between mathematics, development and business knowledge) to an image that would be a Swiss army knife.
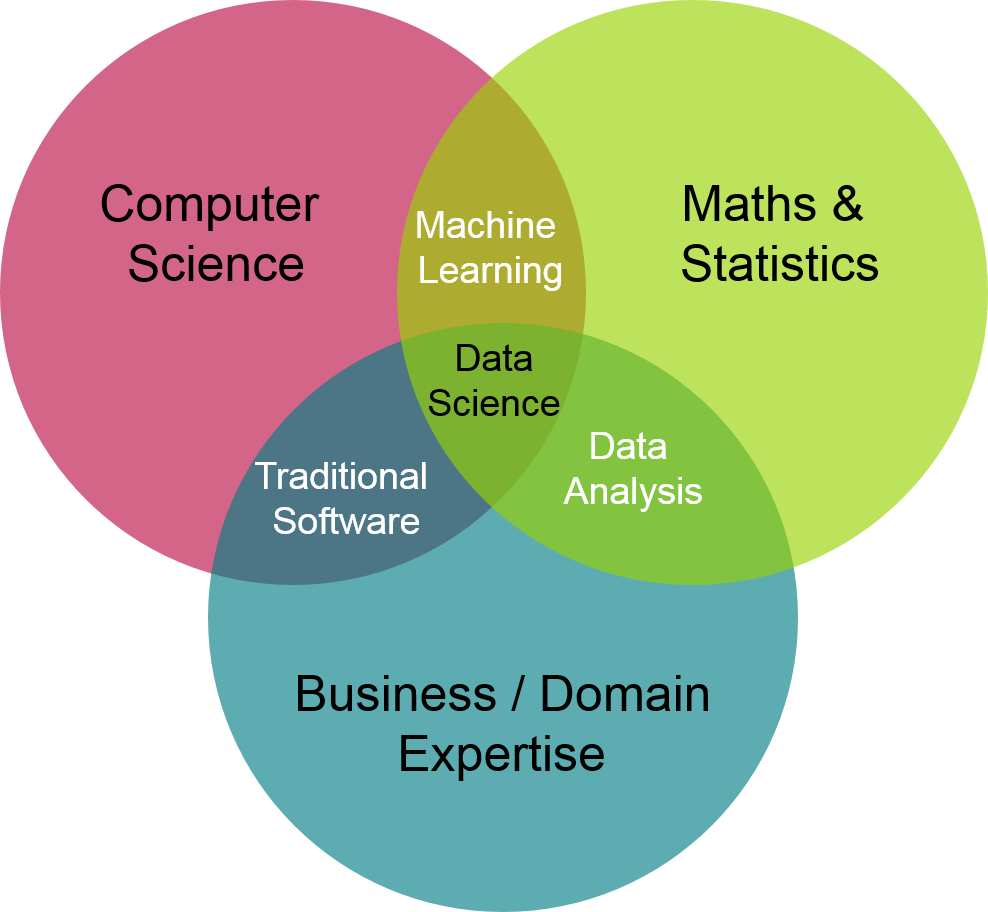
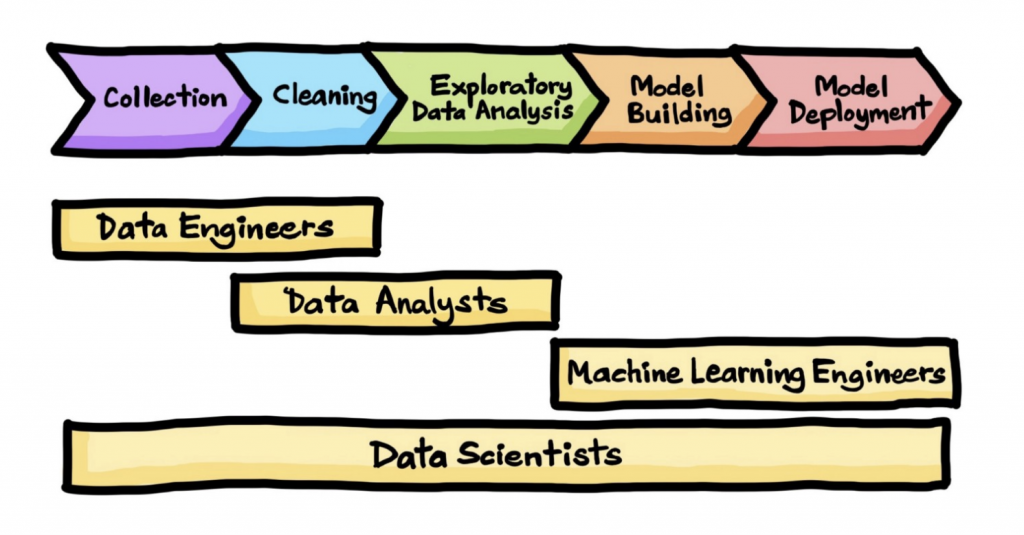
Originally, Data Scientist is a person who will be able to do modelling, by mobilizing business, mathematical and statistical knowledge, using computer support.
However, in the new representations of the profession, it seems that the Data Scientist also takes on the role of Data Engineer, Data Analyst and DevOps.
It is from this observation that Daoud wanted to dig into the evolution of the Data Scientist profession and understand this change of conception.
How did we go from a specialist’s vision, with a Data Scientist focused on research and prototyping, to a vision of a generalist profession that encompasses everything, saving ourselves the care of surrounding ourselves with other specialists such as a Data Analyst, Data Engineer or Machine Learning Engineer?
Originally, the Data Scientist has a core business which is machine learning, modelling and prototyping. This expertise can also be extensive in data analysis and engineering.
However, we can easily draw a line between what is rather the responsibility of a Data Scientist, a Data Engineer and a Data Analyst. On a given expertise, there is not any doubt about which profession has what responsibility, even if the borders flow into each other.
The tasks can therefore easily be distributed on a project between these different specialists.
On the other hand, the border on the devOps side is less clear: Deployment, production, creation of a CI/CD pipeline,… It’s more complicated to say what the Data Scientist and Machine Learning Engineer (MLE) are responsible for. And again, if we agree on the definition of Machine Learning Engineer. For some, a MLE is a data scientist who does production. For others, it is a devOps that works in the machine learning environment. And there are even some who consider that we should no longer say Data Scientist, but Machine Learning Engineer.
The nature of the boundaries between these two roles is not the same. In certain cases there are clear borders, certainly porous, but delimited. In others, they are not as easily defined because we do not know how far the Data Scientist must go and when the MLE takes over. Especially since the Data Analyst and Data Engineer professions are well established, and that MLE is more recent.
Although we do not really know where the limits of Data Science are, we know that there is a devOps part that is the responsibility of the MLE. And it is precisely on this DevOps part that more pressure lies today. The production stage is where projects encounter the most pitfalls and seems to be the most critical today.
On a personal level, as a consultant, Daoud was also able to see that the clients’ reinsurance point had shifted. It was no longer ‘Am I able to predict this based on that?’, but ‘Knowing that I think we can predict this based on that, how do I then deploy it and how will it fit into my IS?’.
On the one hand, it proves that customers are increasingly mature and aware of the potential of their data. But it also corresponds to an inflation of the scope of the Data Scientist. And it also contributes to putting tension on Infrastructure and devOps issues.
This view is also correlated with another fact, described in this article that talks about the technical debts hidden in machine learning systems. During deployment in production, there are indeed issues specific to machine learning and artificial intelligence to consider. This suggests that the person making the model should have the characteristics of the production environment in mind, to avoid this technical pitfall.
However, there is a lack of maturity among Data Scientists on these issues, which is another problem entirely.
What are the prospects for the Data Scientist profession?
How can the arrival of these new issues and the role of Machine Learning Engineer have an impact on the role of Data Scientist?
One possible opinion is that Data Scientists will have to integrate new MLOps skills. But this isn’t the end-all answer to the question.
How could the MLE profession be defined?
We can confidently say that MLE is a growing profession, with a lot of pressure riding on it and with a strong need in the market.
But is the job of MLE a branch that is born from the job of Data Scientist by being a separate profession, or is MLE the new name of the job of Data Scientist?
To fully answer this question, we need to understand who the MLE are in terms of profiles.
We can rely on a study conducted by LinkedIn and published in 2022 on the most growing professions between 2017 and 2021 in the United States. The profession of Machine Learning Engineer appears in fourth position. We also see the original jobs of people who apply for these ads, mostly including developers, Data Scientists and artificial intelligence specialists.
The fact that there are Data Scientists who are part of today’s MLEs, still gives the impression that we are dealing with a split in the Data Scientists profession between different sets of expertise.
We are therefore tempted to think that in data science it is a profession that is splitting in two, with one part taking on certain responsibilities and another taking on others.
To define these responsibilities, we can refer to this diagram:
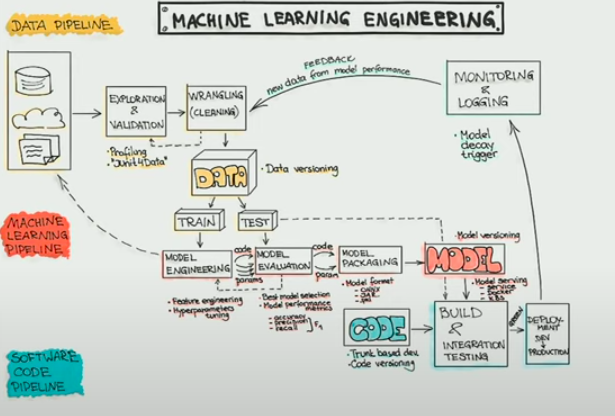
If we are just talking about expertise, we see there is a part providing data and ensuring the availability and logistics of this data. All within actionable and accessible data delivery. This could correspond to the role of the Data Engineer.
We also see there is expertise needed on the production side of models. And that on the machine learning part, the expected deliverable is a model. This could correspond to the role of the Data Scientist.
Lastly, we can make out a third expertise which, from this model, produces code with a test & build part. Starting from the code that contributes to producing a model, we will use application codes to integrate the model and put it into production.
The pressure on the job of Data Scientist comes from the fact that they are asked more frequently to manage this third expertise. While it could easily fit the role of the MLE.
Finally, this diagram and each expertise on it, represent quite well where the different professions will stabilize, what will be expected of each role and where the relay between the role of Data Scientist and MLE is located. The trades will then specialize again because you cannot ask someone to master the entire chain.
To further confirm this conviction, Daoud conducted an analysis of 80 job offers, including 40 Data Scientist offers and 40 MLE offers. For each position, 20 offers on Welcome to the Jungle and 20 offers on LinkedIn were observed.
For each role, the percentage of these offerings that mentioned competence in data engineering, data analysis, business understanding and devOps was analysed.
What expertise for the Data Scientist and MLE professions?
The following results were obtained:
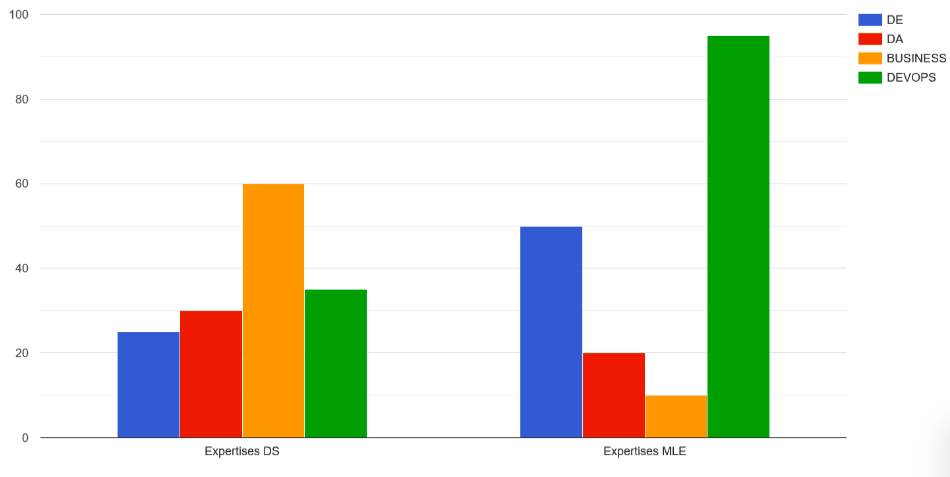
For Data Scientist offers, the distribution of skills sought is:
– Data engineering for 25% of ads,
– Data analysis for 30% of ads,
– Business understanding for 60% of ads — In most of cases, we will consider that for a Data Scientist, it is essential that he knows or has an appetite for the sector in which he works.
– DevOps for 35% of ads,
– And machine learning skills in all ads.
We see that a Data Scientist is someone who knows the data and who is asked to take an interest in the business and to have a good understanding of it.
For MLE offers, the distribution of skills sought is:
– Data engineering for 50% of ads,
– Data analysis for 20% of ads,
– Business understanding for 10% of ads,
– DevOps for 95% of ads.
These results suggest that the MLE arrives in a company where we already know how to predict, or that is reassured about its ability to do so, or even that already has an existing model. The MLE is therefore expected to put into production and ensure the life cycle of the model.
But do you also need to have real machine learning skills as MLE? The short answer is YES, at least to be able to evaluate the model, albeit not very thoroughly. At a minimum they should be knowledgeable about how to monitor the model. After how the performance of the model is measured depends on how it will predict and what we want to predict. It is therefore the responsibility of the Data Scientist to define the right KPI. After which monitoring can be conducted by the MLE.
To conclude, at least in the minds of those who recruit Data Scientists and MLE, it is not the same expertise that is being sought.
Data scientists need to master business expertise to make predictions. They will therefore not be replaced by MLEs, from whom this competence is not particularly expected.
These are two complementary roles, and it’s not expected from either role to know how to do what the other one does.
The difference between an MLE and a Devops can also be subtle, because it mobilizes the same expertise.
The difference is that the MLE will not only version code, but it will also version a model and data. In addition, what is in production does not have deterministic behaviour and is not tested in the same way. It puts into production something that works today, but not necessarily in the long term. Overall these things justify the differences between the two professions in the world of Data.
Finally, it is a sign of market maturity to be able to distinguish more astutely the different professions of the world of Data.
We regularly publish articles on topics of web and mobile product development, data and analytics, security, cloud, hyperautomation and digital workplace.
Follow us on Medium to be notified of the next articles and carry out your professional watch.
You can also find our publications and news via our newsletter, as well as our various social networks: LinkedIn, Twitter, YouTube, Twitch and Instagram.
Want to know more? Check out more of our website and our job offers.